Are Large Language Models (LLMs) the Future of AI?
LLMs: The future of AI? Explore their potential for revolutionizing NLP, creativity, and more.

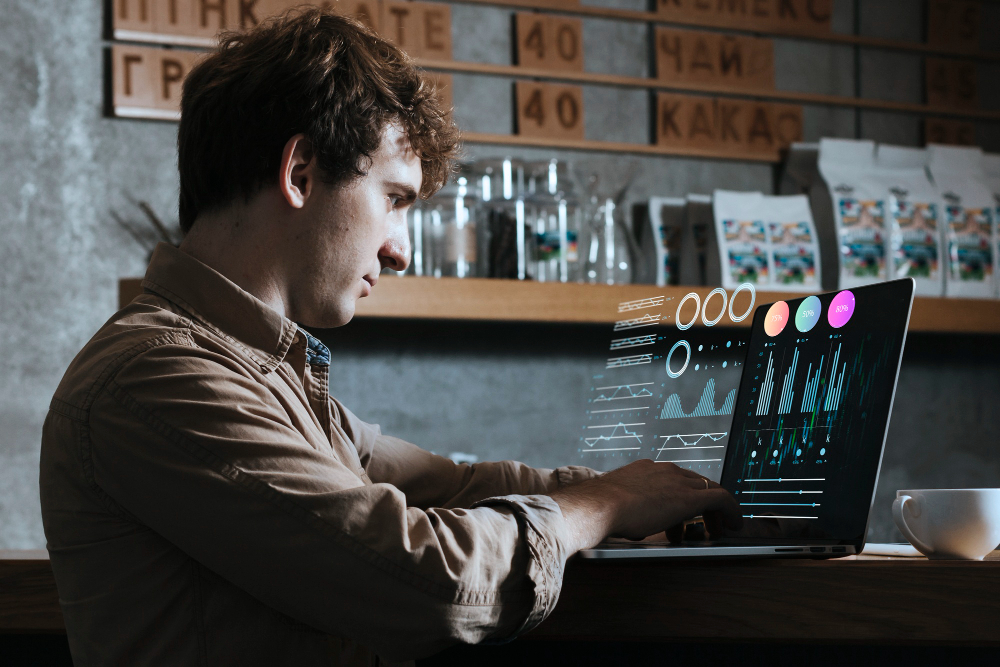
The field of artificial intelligence (AI) has witnessed a remarkable surge in recent years, with Large Language Models (LLMs) emerging as one of the most transformative technologies.
Current projections from Pragma Market Research show how many large language models will be used by 2025. The Global LLM market is also predicted to experience a significant expansion by 2030.

In this post, we will explore how LLMs work, their evolution, use cases across industries, and the different considerations involved in using this technology. Let’s dig deeper!
What are Large Language Models (LLM)?
Large Language Models (LLMs) are AI models trained on massive amounts of text data to understand and generate human language. By learning patterns, grammar rules, and context from vast datasets, LLMs can comprehend the meaning of text, even if it's phrased differently or contains errors.
Additionally, they can generate new text, such as articles, stories, code, or translations. LLMs are highly adaptable and can be fine-tuned for specific tasks, making them versatile tools in various fields.
Large Language Models (LLMs) are trained using a huge amount of data, which allows them to tackle many different tasks effectively. This is a big change from the old way of creating and training separate models for each specific job, which can be expensive, slow, and not as effective.
LLMs significantly advance natural language processing (NLP) and artificial intelligence (AI). Many businesses now rely on this technology to harness AI's power in their work.
Examples of LLMs include:
- GPT-3: One of the most well-known LLMs developed by OpenAI.
- LaMDA: Google's language model for dialogue applications.
- BERT: A language model developed by Google AI.
Read more on Is Chatgpt Generative AI? (How to Make the Best of it)
Types of Large Language Models
Large Language Models (LLMs) can be categorized based on training data, architecture, and intended use cases. Here are six of the main types of LLMs.
- Zero-shot model: This is a large language model trained on a diverse dataset, making it versatile for many applications. It can perform well on tasks it hasn't been explicitly trained on, such as GPT3.
- Fine-tuned model: This includes fine-tuning a zero-shot model like GPT3 on a specific domain, such as programming, to create a specialized model like OpenAI Codex.
- Machine Translation LLMs: These models are designed to translate text from one language to another. Examples include Google Translate and DeepL.
- Question Answering LLMs: These models are trained to answer questions based on a given text corpus. Examples include BERT and T5.
- Transformer-Based LLMs: Most modern LLMs are based on the transformer architecture, which is particularly effective for processing sequential data like text. Examples include GPT-3, BERT, and T5.
- Language Representation Model: Google’s BERT is a prominent example of LRM. It uses deep learning and transformer architectures for language processing tasks.
History and Evolution of LLMs
While their recent surge in popularity might suggest a sudden emergence, LLMs have been developing for years, with companies like Makebot.ai pioneering their use to enhance natural language understanding (NLU) and natural language processing (NLP) capabilities.
Early Days: Chatbots and Rule-Based Systems (1960s)
- ELIZA (1966): The first chatbot, developed by Joseph Weizenbaum at MIT. It simulated conversation using pattern matching and substitution, creating the illusion of understanding.
- DOCTOR: A variation of ELIZA designed to mimic a Rogerian psychotherapist.
Rise of Recurrent Neural Networks (1980s)
- RNNs: Introduced in 1986, RNNs were the first neural networks capable of processing sequential data. They could remember previous inputs and answer questions based on context. However, they suffered from the "vanishing gradient" problem, limiting their ability to retain long-term dependencies.
Rise of Long Short-Term Memory (1990s)
- LSTMs: Introduced in 1997, LSTMs were a specialized type of RNN that addressed the vanishing gradient problem. They used gates to control the flow of information, allowing them to remember information over log sequences.
Gated Recurrent Network (2010s)
- GRUs: Introduced in 2014, GRUs were a simplified version of LSTMs. They used fewer gates, making them more computationally efficient.
Rise of Attention Mechanism (2014)
- Attention: The attention mechanism allowed models to focus on different parts of the input sequence dynamically, improving their ability to capture long-range dependencies.
The Invention of Transformers (2017)
- Transformers: Introduced in 2017, transformers relied solely on attention mechanisms to process sequences. They could process sequences in parallel, making them more efficient than RNN-based models.
Emergence of Large Language Models (2018-onwards)
- BERT (2018): The first large language model, BERT, was pre-trained on a massive amount of text and could be fine-tuned for various tasks.
- GPT-2 (2019), GPT-3 (2020), and others: These models continued to push the boundaries of language modeling, achieving impressive results on a wide range of tasks.
Use of Large Language Models Across Industries
Let's explore how LLMs are transforming various sectors and shaping the future of technology.
Retail and Online Shopping
Large language models (LLMs) significantly impact the retail and e-commerce industries. These AI-powered tools are often integrated into chatbots to enhance customer experiences and drive sales.
LLMs can guide customers through shopping, asking relevant questions to help them find the perfect products. They assist with adding items to carts, selecting payment methods, and completing checkout, reducing cart abandonment and increasing sales. In fact, studies found that 91% of customers appreciate personalized recommendations.
Tools like Frequentli.ai enhance customer interaction with innovative and integrated widgets. Frequentli.ai combines dynamic FAQs and knowledge bases, a powerful self-service tool on landing pages.
Customers can ask questions about products directly on the website, allowing them to find answers instantly without contacting support. This improves customer satisfaction and reduces the workload for support teams.
Healthcare
Large language models (LLMs) are also revolutionizing the healthcare industry by automating tasks, improving efficiency, and enhancing patient care. They can facilitate Administrative Efficiency by handling routine tasks like scheduling appointments, answering patient inquiries, and providing information about services.
LLMs can streamline documentation by automatically gathering, storing, and analyzing patient data. By analyzing patient data, LLMs can help make more accurate diagnoses and recommend appropriate treatments.
Studies have shown that LLMs can achieve an accuracy rate of 83.3% in analyzing historical data and similar cases.
Education
Large language models (LLMs) are transforming learning by offering personalized education and streamlining school administrative tasks.
LLMs can help teachers design learning plans that fit each student’s unique strengths and needs. These models can create customized tests and quizzes that help students practice specific topics.
Research from Knewton, who focuses on personalized learning with the power of AI, shows that students who use LLM-based tutoring can boost their test scores by up to 62%.
LLMs can also automatically grade assignments, tests, and quizzes, giving students quick feedback and saving teachers time.
Finance
LLMs help banks stay updated on the latest rules and best practices, ensuring they follow regulations and reduce risks. These models can also help with compliance tasks, like generating required documents and providing guidance on regulations.
LLMs can also analyze payment histories to spot fraudulent activities, such as unauthorized transactions or identity theft. They can notify customers about suspicious activities, enabling them to take quick action to protect their accounts.
It does not stop there. LLMs can also offer personalized advice on investments, insurance, and retirement planning, helping customers make better financial choices. For example, a recent study found that 60% of Bank of America's clients use LLM-based tools like Erica for financial advice.
Marketing
Lastly, large language models (LLMs) are changing the advertising and marketing industries by smoothing processes, boosting efficiency, and providing personalized experiences.
LLMs can produce engaging articles, social media posts, and blog entries that help promote products and connect with customers. Tools like ChatGPT or Grammarly offer real-time feedback on writing style, spelling, and grammar, ensuring the content is polished and professional.
They can also create personalized content tailored to individual customer preferences and provide customer support systems , especially tools like Frequentli.ai and Prompteam.ai , to gather leads into the marketing campaigns' performance.
LLMs: A Comprehensive Survey of Applications, Challenges, Datasets, Limitations, and Future Prospects
Hadi, M. U., Al-Tashi, Q., Qureshi, R., Shah, A., Muneer, A., Irfan, M., Zafar, A., Shaikh, M. B., Akhtar, N., Al-Garadi, M. A., Hassan, S. Z., Wu, J., & Mirjalili, S. (2015). LLMs: A comprehensive survey of applications, challenges, datasets, limitations, and prospects.
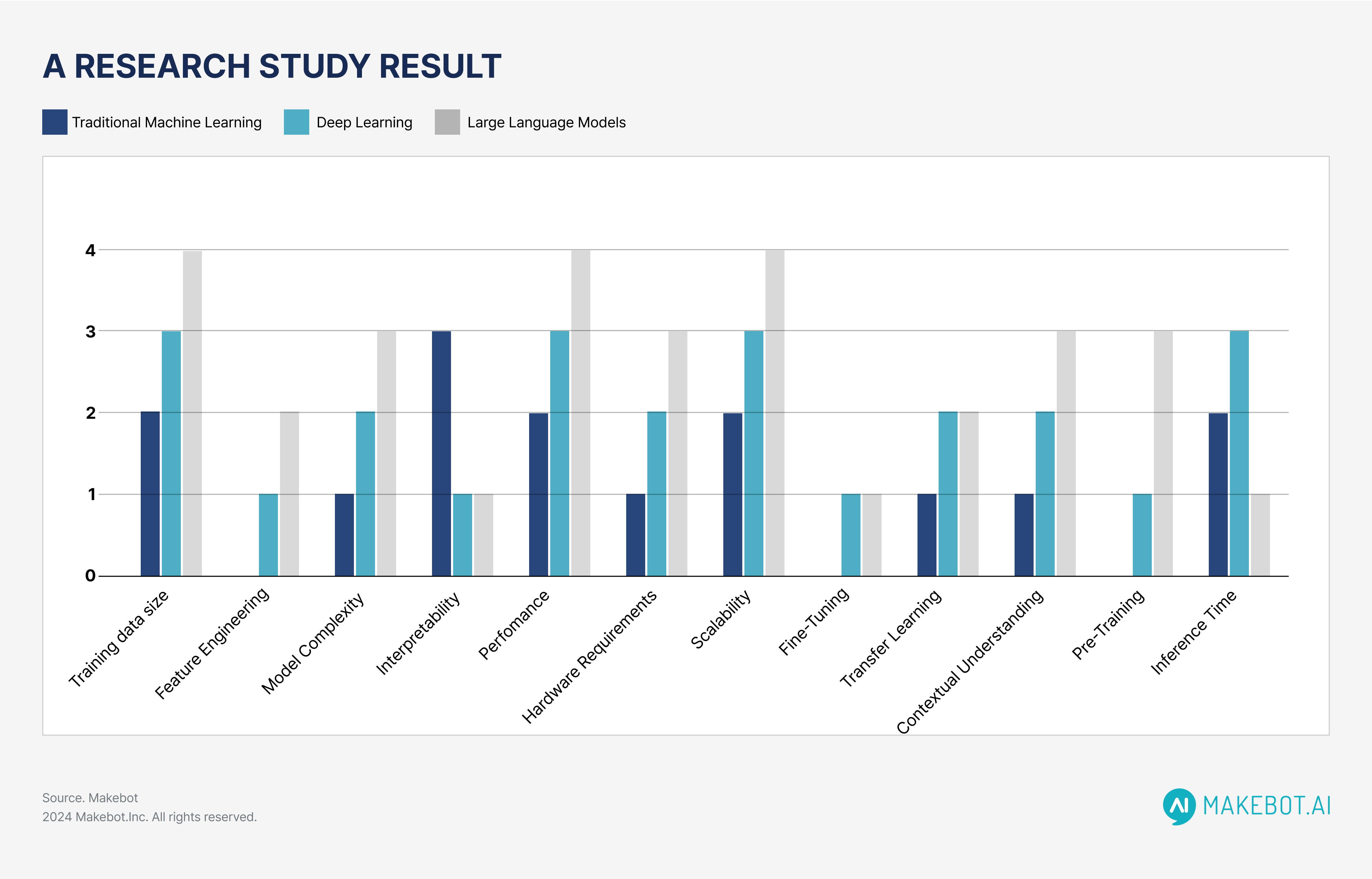
Large Language Models (LLMs) have revolutionized natural language processing by leveraging transformer architectures to achieve remarkable performance in tasks such as text generation, translation, and summarization.
A recent comprehensive survey by Hadi et al. (2015) provides a valuable overview of LLM applications, challenges, datasets, limitations, and prospects. The study highlights the significant advancements achieved by LLMs in recent years, with models like GPT-3 showcasing up to 175 billion parameters.
However, they also argued that LLMs present significant challenges, including ethical concerns related to algorithmic bias and the need for model interpretability.
Training and running large LLMs are also substantial computational resources. Additionally, LLMs can become overspecialized, limiting their ability to generalize to new tasks.
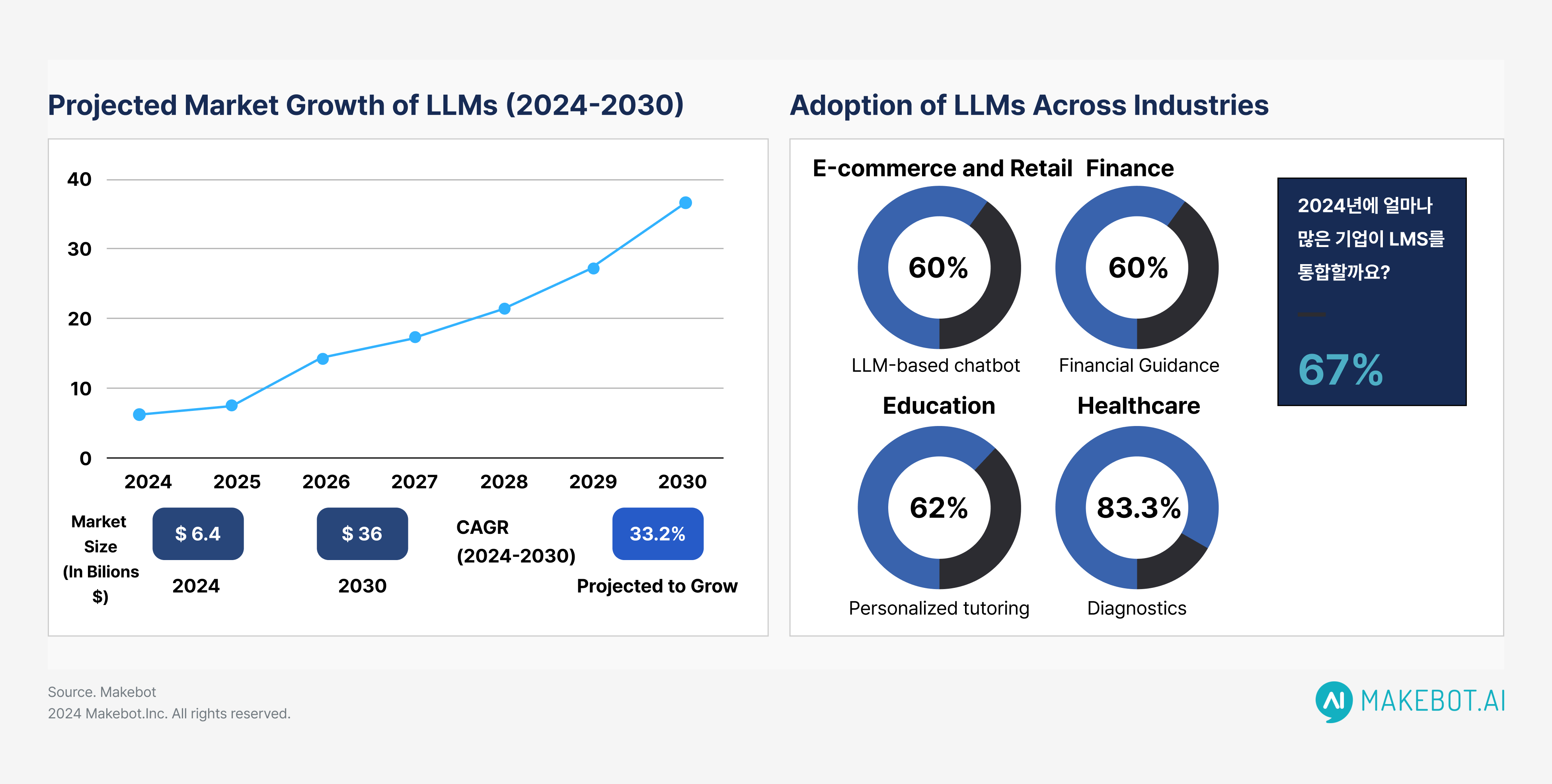
What Can We Expect in the Future?
Massive Market Growth and Value
The LLM market is forecasted to hit $36 billion by 2030, growing at a CAGR of 33.2%. North America will capture $105.5 million of this market by 2025, while the Asia Pacific region is projected to experience the fastest growth. LLMs are expected to support over 750 million applications globally by 2025.
Increasing Business Adoption and Efficiency Currently
67% of businesses use LLMs, and by 2028, 85% are predicted to rely on them for tasks like content generation and customer service, leading to a 40% reduction in operational costs. Despite this, only 23% have fully implemented LLMs due to concerns around privacy and ethical AI.
Accuracy and AI-Driven Personalization
LLMs are set to reach over 90% accuracy in tasks like diagnostics and customer interactions. In education, personalized learning powered by LLMs improves student test scores by 62%. In e-commerce, 91% of customers appreciate personalized recommendations generated through LLMs, enhancing business cross-sales.
Ethical AI and Transparency
By 2026, over 70% of LLM applications will include bias mitigation and transparency measures, ensuring responsible use. With healthcare LLMs achieving 83.3% diagnostic accuracy, ethical AI becomes vital in safeguarding patient data and ensuring fair treatment outcomes.
More on LLM Applications in Generative AI Explained: Create Anything with AI in 2024
Closed vs Open-Source LLMs
Open-source large language models (LLMs), like GPT-NeoX and BLOOM, are popular for their high customization and transparency, preferred by up to 90% of developers. They are also cost-effective, reducing deployment costs by up to 80%. However, they are often less secure compared to closed-source models.
Closed-source LLMs, such as GPT-4 and Google Bard, offer 85% better security and are 50% more scalable, making them ideal for enterprises, but they come with higher costs due to subscription fees and support services.
Experience LLM Potential through Chatbots
Large Language Models are undoubtedly shaping the future of AI, but for many businesses, the path to implementation can seem daunting. Here's where chatbots come in – a practical and user-friendly way to experience the power of LLMs.
Makebot.ai builds custom chatbots to address your needs and deliver exceptional customer service, helping your business flourish in conversational AI. Talk to us today!