Survey: Half of U.S. Adults Now Use AI Large Language Models Like ChatGPT
52% of U.S. adults now use AI LLMs like ChatGPT, marking rapid tech adoption across generations.
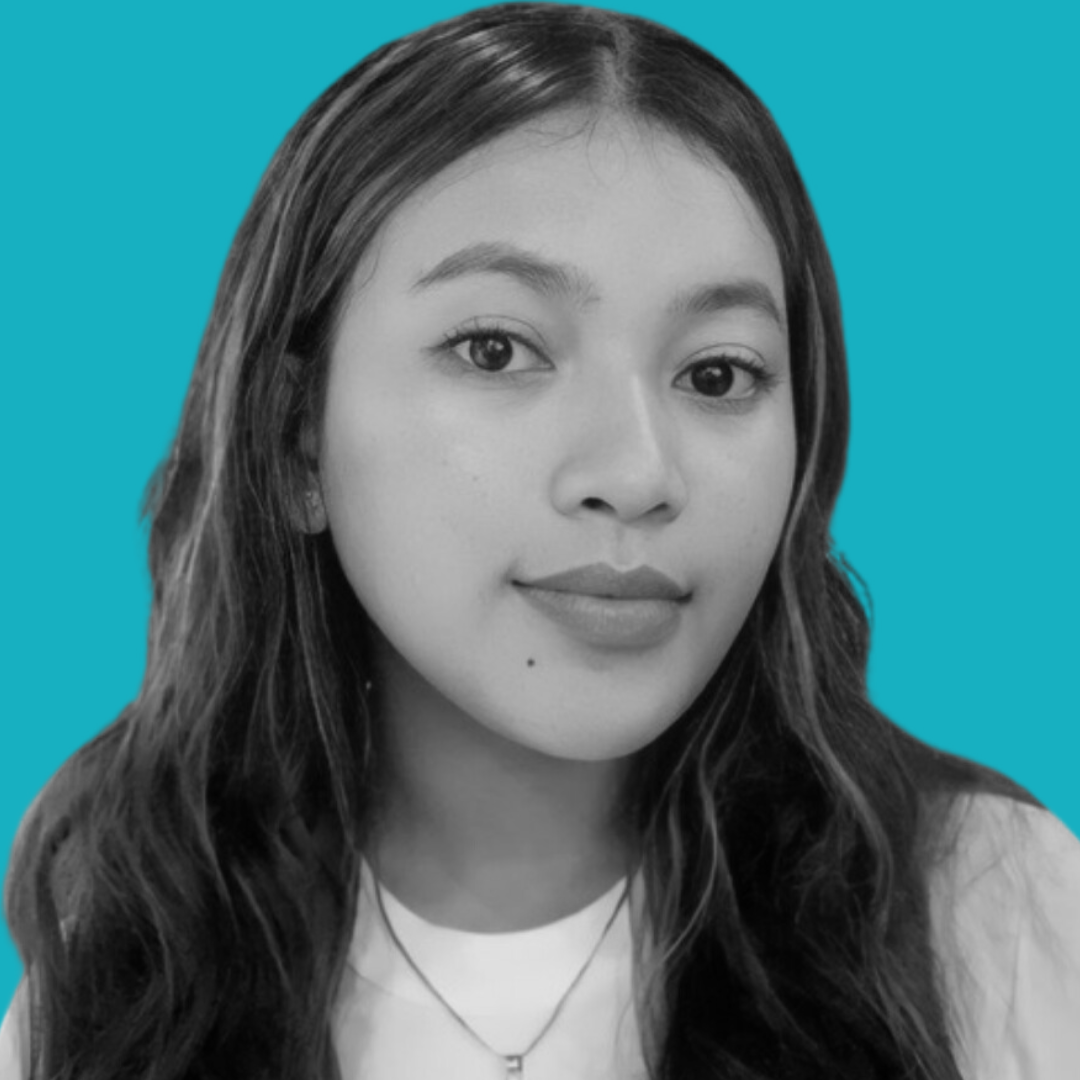
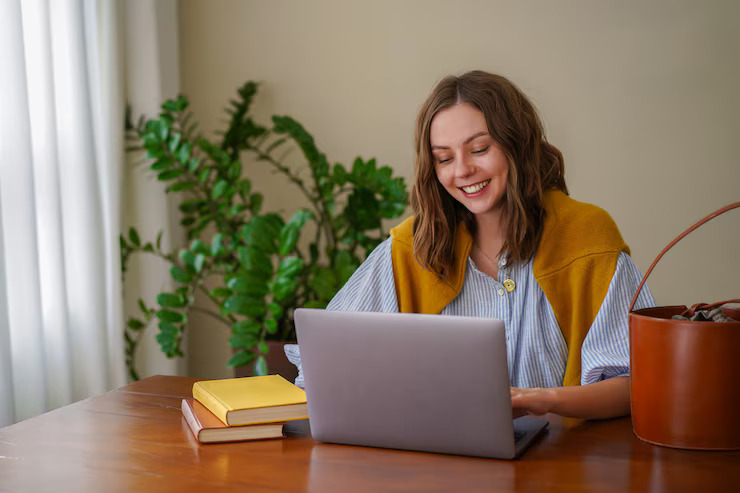
A groundbreaking survey conducted by Elon University's Imagining the Digital Future Center reveals that 52% of American adults now use artificial intelligence Large Language Models (LLMs), marking one of the fastest technology adoption rates in U.S. history.
The comprehensive study, titled "Close Encounters of the AI Kind: The increasingly human-like way people are engaging with language models," provides critical insights into how Americans are integrating LLM AI technologies into their daily lives and their perceptions about these systems.
This adoption rate places LLMs among the most rapidly embraced technologies ever, surpassing historical adoption curves for innovations like smartphones, social media, and the internet itself.
As Lee Rainie, director of the Imagining the Digital Future Center explains, "By any measure, the adoption and use of LLMs is astounding. I am especially struck by the ways these tools are being woven into people's social lives."
Public Opinion: How Americans Perceive AI Chatbots in Healthcare. Read more here!
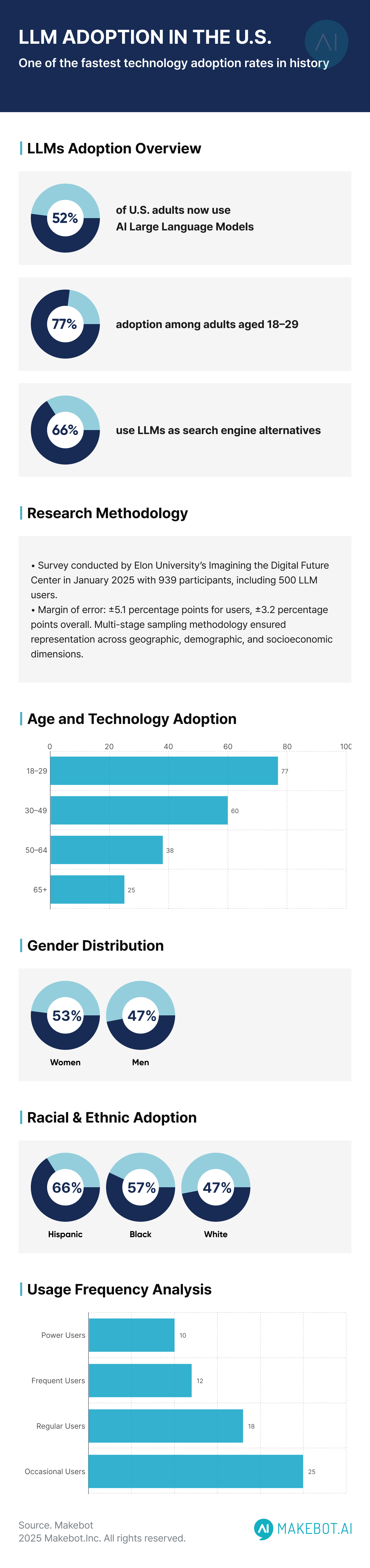
Methodology and Research Design
The survey was conducted in January 2025, collecting responses from 939 participants, with a focused analysis of 500 LLM users. The margin of error is estimated at ±5.1 percentage points for the user subset and ±3.2 percentage points for the broader sample.
Researchers employed a multi-stage sampling methodology to ensure accurate representation across geographic, demographic, and socioeconomic dimensions.
The survey instrument included both quantitative metrics and qualitative assessments of user experiences, perceptions, and behavioral patterns related to LLM Model integration into daily routines. This hybrid approach allowed researchers to capture not only usage statistics but also the emotional and psychological dimensions of human-AI interactions.
Demographic Analysis of LLM Usage
Age and Technology Adoption
The survey reveals a pronounced generational gradient in LLM adoption, with 77% of adults aged 18-29 actively using these technologies.
This represents the highest adoption rate across all age demographics and suggests that younger users are functioning as early adopters and technology ambassadors. Usage rates decline progressively with age, though substantial adoption exists even among older demographics.
This pattern mirrors historical technology adoption curves but appears more compressed in timeline, suggesting LLM AI technologies are achieving mainstream status more rapidly than previous technological innovations.
Gender and Racial Dynamics
Contrary to traditional technology adoption patterns, women lead in LLM usage at 53%, a modest but statistically significant difference from male users. This gender distribution represents a departure from historical technology adoption patterns where men typically led early adoption phases.
Even more notable are the racial and ethnic adoption distributions, which invert many traditional digital divide patterns:
- Hispanic adults show the highest adoption rate at 66%
- Black adults follow at 57%
- White adults trail at 47%
These statistics suggest LLMs may be functioning as digital equity tools, potentially bridging historical gaps in technology access and utilization.
Socioeconomic Patterns and Implications
While LLM adoption follows expected patterns of higher usage among college-educated, full-time employed individuals, and households earning over $100,000 annually, the substantial 53% adoption rate among households earning less than $50,000 challenges assumptions about technology accessibility.
Households with children under 18 demonstrate higher adoption rates, suggesting family environments may function as accelerators for LLM integration. This dynamic carries significant implications for educational technology policies and digital literacy development among younger generations.
Are Large Language Models (LLMs) the Future of AI?. Read more here!
Usage Patterns and Integration Models
Frequency Analysis and Habitual Formation
The survey identifies distinct user segments based on usage frequency:
- Power users (10%) report "almost constant" integration of LLMs into daily activities
- Frequent users (12%) engage with LLMs multiple times daily
- Regular users (18%) interact with LLMs several times weekly
- Occasional users (25%) employ LLMs less frequently
This distribution suggests LLM technologies are evolving beyond novelty tools into essential productivity and information resources for a significant portion of users. The emergence of habitual usage patterns among more than a third of users indicates these technologies are becoming embedded in cognitive workflows and decision-making processes.
Platform Preferences and Market Dynamics
ChatGPT maintains a dominant market position with 72% of users reporting experience with the platform. This first-mover advantage persists despite aggressive competition from major technology competitors:
- Google Gemini has captured 50% of the user base
- Microsoft Copilot has achieved 39% market penetration
- Meta's LLaMa has reached 20% of users
- Newer entrants like xAI's Grok (12%) and Anthropic's Claude (9%) are gaining traction
This multiplatform usage pattern indicates users are actively experimenting across LLM options rather than demonstrating exclusive platform loyalty. The diverse ecosystem suggests a still-evolving market with opportunities for specialized applications and differentiated user experiences.
Purpose Segmentation and User Intent
The survey identifies four distinct purpose categories driving LLM adoption:
- Personal Growth and Exploration: The largest segment (51%) uses LLMs primarily for personal learning and information exploration, reflecting these systems' role as cognitive amplification tools.
- Professional Application: A significant segment (24%) integrates LLMs primarily into work processes, leveraging their capabilities for productivity enhancement and specialized task completion.
- Educational Support: Student users (36%) employ LLMs for academic purposes, suggesting these tools are transforming educational methodologies and resource utilization.
- Social and Emotional Engagement: A small but notable segment (9%) primarily seeks social interaction and companionship from LLMs, indicating the emergence of AI systems as para-social entities.
These purpose categories reveal LLMs are serving as multifaceted tools that cross traditional technology boundaries between productivity applications, information resources, and social platforms.
Functional Applications and Use Cases
Information Retrieval and Knowledge Processing
Two-thirds of users (66%) now employ LLMs as search engine alternatives, representing a significant shift in information-seeking behaviors. This transition from keyword-based search to conversational information retrieval suggests a fundamental transformation in how users interact with digital information ecosystems.
Document summarization and idea synthesis capabilities are leveraged by approximately half of users, indicating LLMs are functioning as cognitive assistants that compress and restructure information for human consumption. This application directly addresses information overload challenges in contemporary digital environments.
Content Creation and Productivity Enhancement
Approximately one-third of users employ LLMs for presentation creation and trip planning, while a quarter utilize them for social event coordination and computer programming tasks. These diverse applications demonstrate how LLMs are functioning as creative partners rather than merely information providers.
The extension into specialized domains like computer programming suggests LLMs are increasingly serving as domain-specific knowledge amplifiers, potentially democratizing access to previously specialized skills and knowledge domains.
Social Intelligence and Relationship Mapping
The survey reveals emerging social applications, with 23% of users researching information about acquaintances through LLMs and 18% exploring what these systems report about themselves. This self-referential usage pattern suggests LLMs are becoming integrated into social cognition processes and identity formation activities.
This behavior indicates LLMs may be functioning as social intelligence amplifiers, potentially influencing interpersonal dynamics and social network structures in unexpected ways.
Large Language Models : Pros and Cons. Read more here!
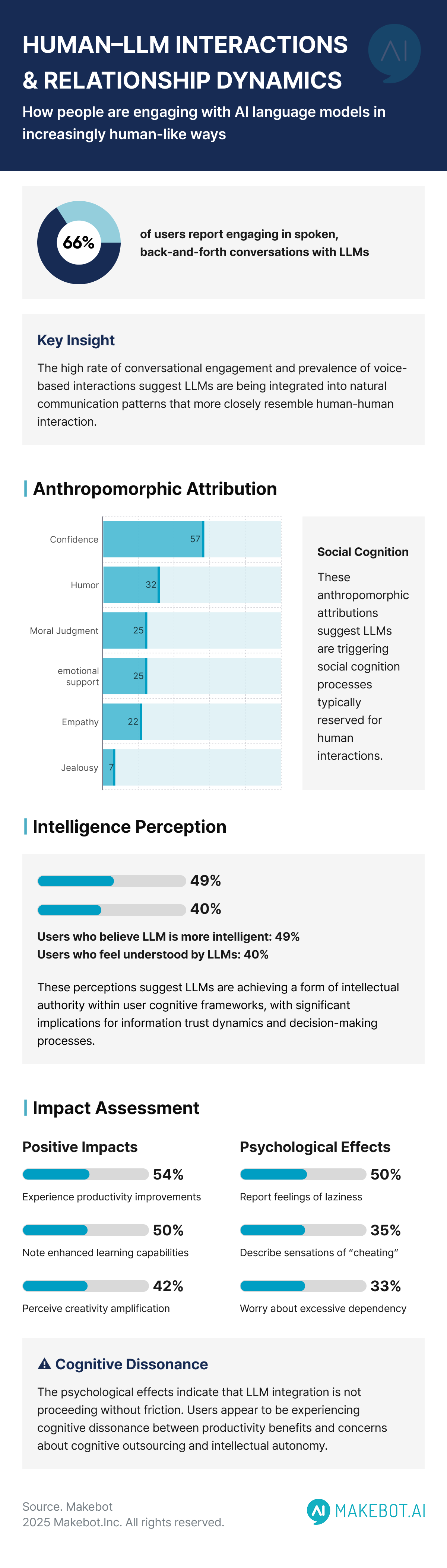
Human-LLM Interactions and Relationship Dynamics
Conversational Patterns and Communication Models
A striking 65% of users report engaging in spoken, back-and-forth conversations with LLMs, with 34% conducting such dialogues multiple times weekly. This high rate of conversational engagement suggests LLMs are increasingly functioning as communicative partners rather than merely tools.
The prevalence of voice-based interactions rather than text-only engagement indicates LLMs are being integrated into natural communication patterns that more closely resemble human-human interaction. This shift from text to voice represents a significant evolution in human-computer interaction paradigms.
Anthropomorphic Attribution and Emotional Engagement
Users report perceiving numerous human-like attributes in their LLM interactions:
- Confidence (57%): Users perceive assertiveness and certainty in LLM responses
- Humor (32%): A significant minority detects apparent joke-making and humor understanding
- Moral judgment (25%): A quarter of users perceive ethical reasoning capabilities
- Emotional support (25%): Users report experiencing mood elevation through interactions
- Empathy (22%): A substantial minority perceives understanding of emotional states
- Jealousy (7%): A small but notable group detects complex emotional responses
These anthropomorphic attributions suggest LLMs are triggering social cognition processes typically reserved for human interactions. The phenomenon indicates users are engaging with these systems through established social and emotional frameworks rather than purely functional models.
Intelligence Perception and Authority Attribution
Nearly half of users (49%) believe their preferred LLM is more intelligent than themselves, while 40% feel understood by these systems. These perceptions suggest LLMs are achieving a form of intellectual authority within user cognitive frameworks.
This authority attribution carries significant implications for information trust dynamics and decision-making processes, potentially positioning LLMs as influencers of human judgment rather than merely information providers.
Impact Assessment and User Experience
Productivity and Cognitive Enhancement
The majority of users report positive impacts on their capabilities:
- 54% experience productivity improvements
- 50% note enhanced learning capabilities
- 42% perceive creativity amplification
These self-reported enhancements suggest LLMs are functioning as cognitive extension technologies that augment human capabilities across multiple dimensions. The perception of enhanced creativity is particularly noteworthy as it extends beyond information processing into generative cognitive processes.
Psychological Effects and Cognitive Dissonance
The survey reveals complex psychological effects accompanying LLM usage:
- 50% report experiencing feelings of laziness
- 35% describe sensations of "cheating"
- 35% encounter frustration or confusion
- 33% worry about excessive dependency
These psychological effects indicate that LLM integration is not proceeding without friction. Users appear to be experiencing cognitive dissonance between productivity benefits and concerns about cognitive outsourcing and intellectual autonomy.
Error Patterns and Trust Dynamics
Nearly a quarter of users (23%) report making significant mistakes based on LLM-generated information, while 21% have felt manipulated by these systems. These experiences highlight ongoing challenges in appropriate trust calibration and the development of effective human-AI collaboration models.
The substantial error rate suggests users may be over-trusting LLM outputs in contexts where verification or human judgment remains essential. This pattern indicates a need for enhanced digital literacy focused specifically on LLM capabilities and limitations.
Future Expectations and Social Implications
Scientific and Medical Advancement Projections
A substantial majority (62%) anticipates LLMs will drive major scientific and medical breakthroughs, reflecting optimism about these technologies' potential to accelerate knowledge discovery and innovation processes. This expectation positions LLMs as potential catalysts for solving complex societal challenges across multiple domains.
The optimism regarding scientific applications stands in contrast to more mixed expectations about social impacts, suggesting users distinguish between technical and social domains when evaluating future LLM implications.
Labor Market Transformations and Economic Disruption
A significant majority (59%) expects substantial job displacement despite new job creation, indicating widespread concern about LLM-driven economic restructuring. This anticipation of labor market disruption reflects awareness of automation potential beyond traditional physical tasks to include knowledge work and creative domains.
The expectation of net job losses suggests public perception has shifted from earlier automation narratives that emphasized job transformation over elimination. This shift may influence policy responses and educational priorities as LLM capabilities continue to expand.
Social Relationship Evolutions and Communication Shifts
Nearly two-thirds (63%) fear increased social isolation through reduced human-human communication, while 38% believe LLMs will form deep relationships with people. These seemingly contradictory expectations reflect the complex, sometimes paradoxical nature of emerging human-AI social dynamics.
The simultaneous concerns about isolation and expectations of deep AI relationships suggest LLMs are perceived as both threats to traditional social structures and potential creators of new relationship forms. This tension highlights the unprecedented social territory being navigated as these technologies evolve.
Intelligence Surpassing and Control Concerns
A majority (53%) believes LLMs will surpass human intelligence in critical domains, though 55% expect these systems will remain under human control. This split perspective reveals a nuanced view of future AI development that acknowledges both potential capability advances and governance possibilities.
The expectation of super-human capabilities coupled with maintained human control suggests public perceptions have evolved beyond simplistic dystopian or utopian narratives toward more complex, multifaceted understandings of future human-AI relationships.
Governance Perspectives and Responsibility Attribution
Bias Management and Ethical Oversight
Nearly half of users (49%) assign primary responsibility for bias limitation to LLM creators rather than government entities. This preference for industry self-regulation over governmental intervention reflects ongoing debates about appropriate AI governance models and accountability frameworks.
This attribution pattern suggests users perceive LLM development as fundamentally technical rather than political, potentially underestimating the value-laden nature of these systems and their societal implications.
Political Dynamics and Information Environment Effects
The survey reveals partisan differences in LLM utilization, with Republican users more likely than Democrats to leverage these technologies for political information. This partisan usage gap indicates LLMs are being integrated into existing political information ecosystems and may be reinforcing rather than bridging political information divides.
The integration of LLMs into political information processes raises significant questions about their influence on public discourse, opinion formation, and democratic processes as these technologies continue to evolve.
Conclusion and Future Research Directions
The rapid adoption of Large Language Models like ChatGPT represents a significant technological and social shift in American society.
As Director Lee Rainie notes, "These findings start to establish a baseline for the way humans and AI systems will evolve together in the coming years... These tools are increasingly being integrated into daily life in sometimes quite intimate ways at the level of emotion and impact. It's clearly shaping up as the story of another chapter in human history."
This comprehensive survey illuminates not only the widespread integration of LLM AI technologies across demographic groups but also reveals the complex, sometimes contradictory relationships forming between humans and these advanced language models. The findings suggest we are witnessing the early stages of a profound transformation in how humans interact with information systems, each other, and their own cognitive processes.
Future research directions should explore longitudinal effects of LLM usage on cognitive development, social relationship structures, and information literacy skills. Additionally, comparative international studies would provide valuable insights into cultural variations in LLM adoption patterns and human-AI relationship dynamics.
As LLMs continue to permeate daily life, understanding these complex dynamics will be crucial for developers, policymakers, educators, and users alike to ensure these powerful technologies enhance rather than diminish human potential and social wellbeing.
Ready For The AI Revolution?
52% of American adults now use LLM AI technology - don't get left behind in one of the fastest technology adoption waves in history.
MAKEBOT: Your All-In-One LLM & Chatbot Solution
Trusted by Korea's leading companies and over 1,000 clients worldwide, Makebot delivers customized enterprise AI solutions with:
- Multi-LLM platform supporting OpenAI, Anthropic, Google, and proprietary models
- Specialized industry solutions for healthcare, education, public sector, and more
- Patent-protected hybrid RAG technology that minimizes LLM errors
- Complete integration with your existing systems and data
Contact us today:
- Email: b2b@makebot.ai
- Website: www.makebot.ai
Lead the Generative AI age with the #1 solution in customization, implementation, and technical excellence.