Recent News: Large Language Models Surpass Human Experts in Predicting Neuroscience Results
LLMs outperform human experts in predicting neuroscience results with 81.4% accuracy rate.

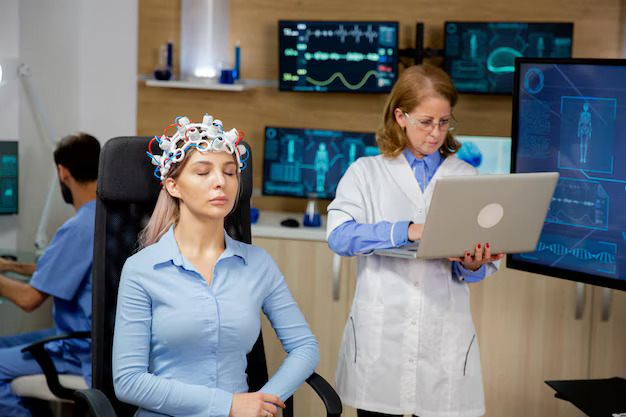
A recent study by University College London (UCL) has demonstrated that large language models (LLMs) outperform human experts in predicting neuroscience research outcomes.
Published in Nature Human Behaviour, the research showed that LLMs achieved higher accuracy rate in distinguishing between real and modified neuroscience abstracts, compared to the accuracy rate of the human experts.
The success of LLMs lies in their ability to synthesize information across entire research abstracts, not just focusing on results.
This breakthrough suggests a future where AI models could assist researchers in designing experiments and predicting outcomes, accelerating the pace of scientific discovery.
Check this out: LLMs in Healthcare: September 2024's Medical Breakthroughs

How Well Do AI Models Perform in Predicting Neuroscience Results?
The research, published in Nature Human Behaviour, set out to investigate whether AI models could predict the results of neuroscience experiments more effectively than human experts.
Researchers created a test called BrainBench, where participants had to distinguish between two research abstracts—one real, and the other modified with plausible but incorrect results.
- LLMs vs. Human Experts:some text
- LLMs achieved an 81.4% accuracy in predicting the correct abstracts.
- Human experts—comprising 171 researchers with an average of 10.1 years of experience in neuroscience—achieved only 63.4% accuracy.
- When limited to the top 20% of most expert human responses, the accuracy only improved to 66.2%.
These results highlight a significant gap between the AI models and human experts in predicting neuroscience outcomes.
More on LLMs here: 10 Healthcare Challenges Solved by AI Chatbots and LLMs
What Makes AI Models So Effective in Neuroscience Predictions?
Data Synthesis Across Multiple Sections
One of the key reasons why LLMs outperformed human experts was their ability to synthesize information across the entire abstract.
While human experts often focused only on the results section—a critical but narrow part of a research paper—LLMs could also integrate insights from the background and methods sections, giving them a more comprehensive understanding of the study's context and findings.
LLMs' Ability to Learn Patterns
Unlike traditional data retrieval systems, LLMs do not simply memorize the content of neuroscience papers.
According to the study, they are able to identify underlying patterns and learn from the data in a way that allows them to forecast experimental outcomes. The team tested the models on 200 human-created abstracts and 100 GPT-4 generated abstracts.
Model Size and Training
Interestingly, smaller models—like those with 7 billion parameters—performed just as well as larger models, suggesting that massive computational power may not be as critical as once believed.
When BrainGPT, a version of the Mistral model fine-tuned with 1.3 billion tokens from over 100 neuroscience journals, was tested, it outperformed its base counterpart with 86% accuracy compared to 83%.
Want to read more regarding LLMs in healthcare? Click here!
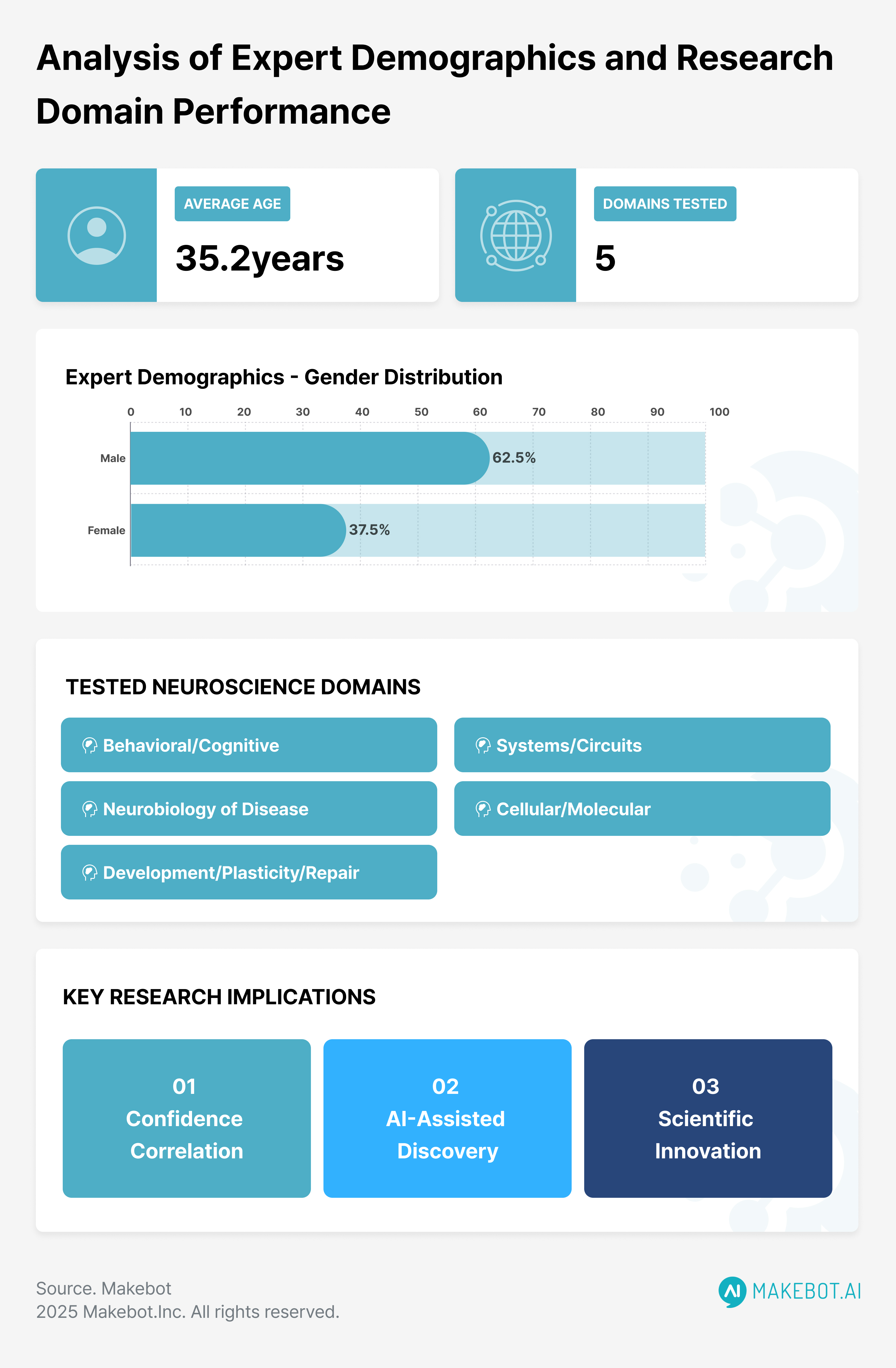
Insights into Human Performance
Despite their expertise, human participants faced challenges in identifying accurate abstracts:
Confidence Correlation
Both humans and LLMs exhibited calibrated confidence in their responses.
The more confident the model or the expert, the more likely their answers were to be correct. However, even the most confident human responses did not surpass AI predictions.
Expert Demographics
Human participants came from diverse backgrounds, including doctoral students, postdocs, faculty, and research scientists.
The majority were male (62.5%), with an average age of 35.2 years.
Despite their expertise, the AI models still outperformed them across all five tested neuroscience domains, including behavioral/cognitive, systems/circuits, neurobiology of disease, cellular/molecular, and development/plasticity/repair.
Implications for the Future of Neuroscience Research
This study raises important questions about the future role of AI in scientific discovery:
AI-Assisted Discovery
“This demonstrates that LLMs can effectively synthesize and interpret complex scientific information in ways that surpass human capabilities,” said Dr. Ken Luo, the lead author of the study.
The team envisions AI models assisting scientists by predicting experimental outcomes, which could accelerate research timelines and inform study designs.
AI and Scientific Exploration
Interestingly, the study's senior author, Professor Bradley Love, remarked,
“What is remarkable is how well LLMs can predict the neuroscience literature. This success suggests that a great deal of science is not truly novel but conforms to existing patterns of results in the literature. We wonder whether scientists are being sufficiently innovative and exploratory.”
Click here to learn more about LLMs in health efficiency!
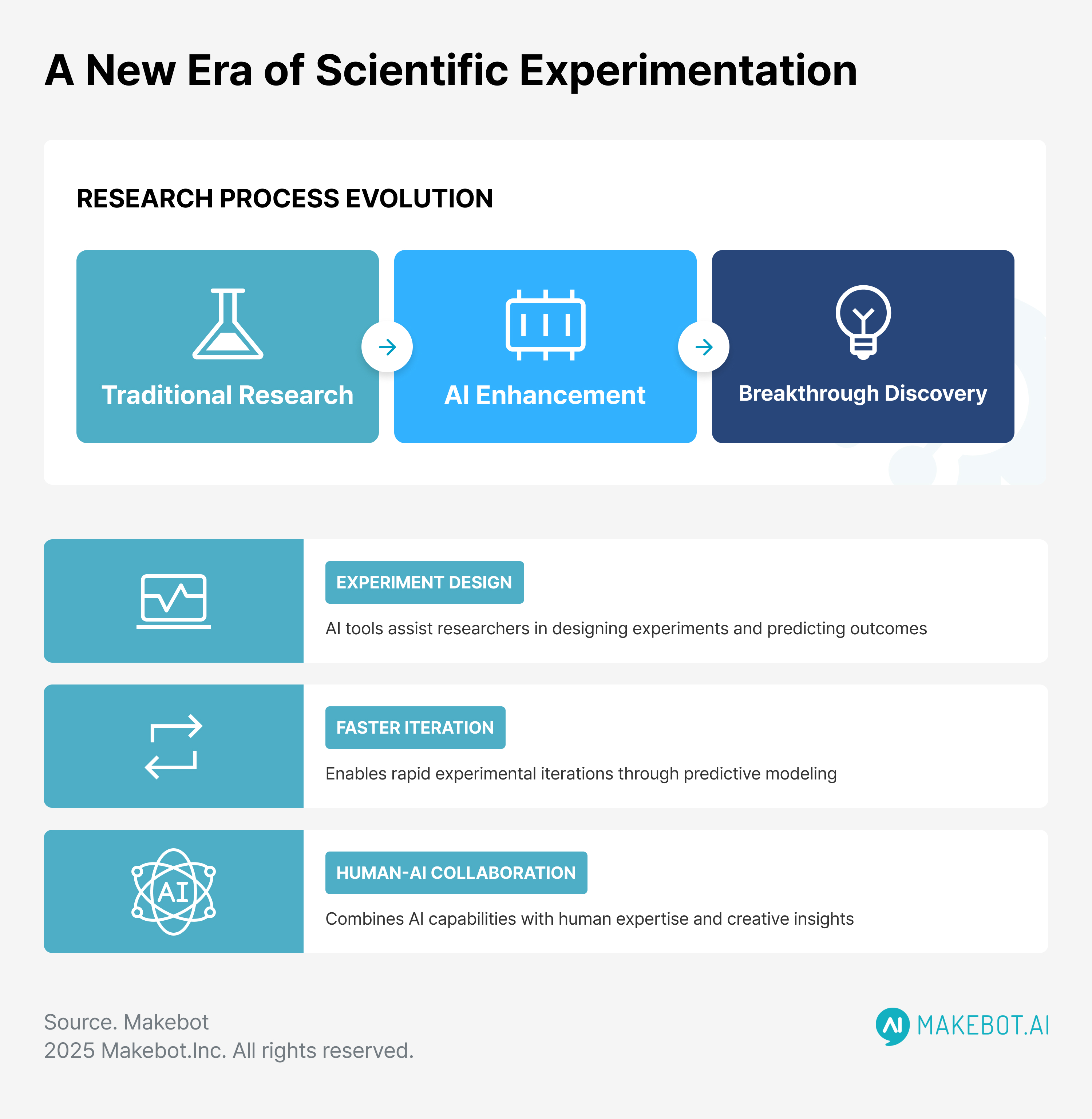
The Future: AI-Driven Research Designs
What’s next for the collaboration between AI and neuroscience?
Future Applications
Dr. Luo envisions a future where AI tools assist researchers in designing experiments and predicting the likelihood of various outcomes.
By inputting experimental designs and anticipated findings into AI models, researchers could receive predictions about the most probable results, enabling faster iteration and more informed decision-making in experiment design.
Broader Impact
This approach, though initially applied to neuroscience, is universal and could be adapted for other scientific fields.
As Professor Love suggests, “It won’t be long before scientists are using AI tools to design the most effective experiment for their question.”
Thus, while LLMs have shown their potential to enhance scientific discovery, human expertise remains invaluable for providing critical context and creative insights.
The future may see a powerful collaboration between AI and scientists, streamlining the process of experimentation and accelerating research breakthroughs.
Revolutionize Your Research with Makebot's AI Solutions
Unlock the potential of AI-driven research with Makebot’s cutting-edge chatbot and LLM solutions. Our advanced models, optimized for a variety of industries, empower researchers to accelerate discovery, predict outcomes, and design experiments faster than ever before.
Ready to transform your research?
Email Us Today (b2b@makebot.ai) to get started and discover how Makebot can help you lead the future of scientific exploration.