Study Suggests Physician's Medical Decisions Benefit from Chatbot Integration
AI chatbots improve physician decisions, enhancing clinical management and patient outcomes.

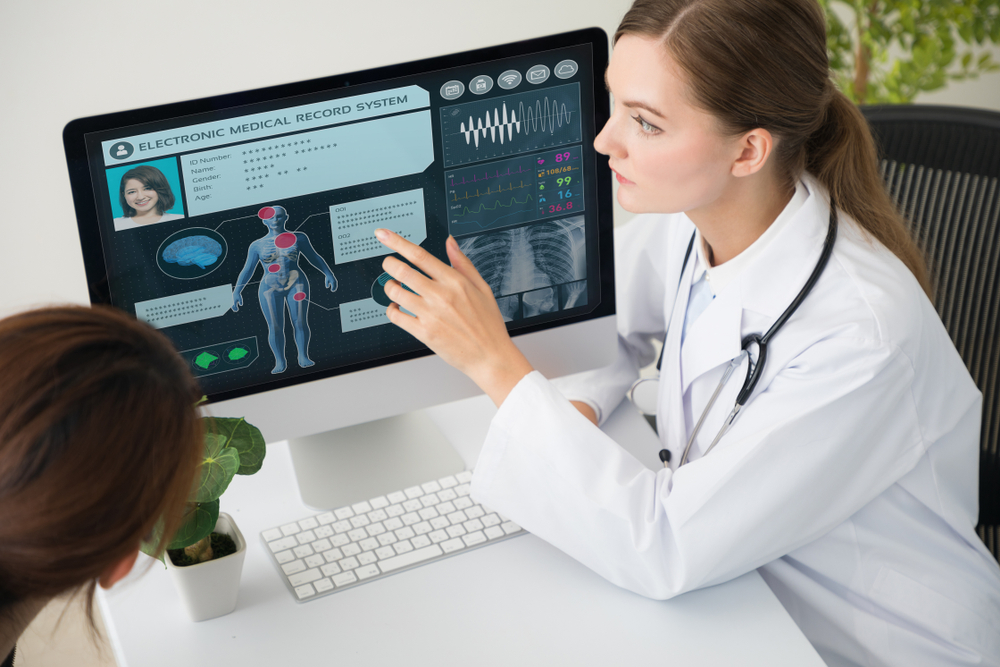
Recent research published in Nature Medicine reveals that AI chatbots are not only advancing in diagnostic capabilities but are also showing significant promise in enhancing physicians' clinical management reasoning—the complex decision-making that follows diagnosis.
This comprehensive study challenges traditional thinking about AI in healthcare and points to a future where human expertise and artificial intelligence collaborate to improve patient outcomes.
Use Cases for Natural Language Processing in Healthcare. Read more here!

Key Findings
The Stanford Medicine study, led by Dr. Jonathan H. Chen and colleagues, evaluated the performance of three distinct groups on clinical management tasks:
- AI chatbots operating autonomously
- Physicians with chatbot assistance
- Physicians using only conventional resources (internet searches and medical references)
The results showed that physicians using chatbot assistance performed significantly better than those relying solely on conventional resources.
Notably, autonomous AI chatbots demonstrated superior performance compared to physicians without AI assistance, but physicians partnered with chatbots achieved comparable results to the AI working alone.
The study involved 92 physicians across multiple regions and institutions in the United States, with 46 physicians in the chatbot-assisted group and 46 in the conventional resources group. When scored against a clinical management rubric developed by board-certified physicians, the chatbot-assisted group achieved significantly higher scores.
Clinical Management Reasoning: Beyond Diagnosis
While previous studies have highlighted AI chatbots' capabilities in disease diagnosis, this research delves into the more nuanced aspects of AI in medicine.
As study co-lead author Dr. Ethan Goh explains, clinical management reasoning involves determining optimal treatment paths after diagnosis—similar to choosing the best route after identifying a destination on a map.
For example, when a physician discovers a sizable mass in a patient's upper lung, they face several possible management approaches: immediate biopsy, scheduling a procedure for later, or ordering additional imaging. The optimal decision depends on numerous factors beyond the clinical presentation alone.
This decision-making process requires weighing multiple factors:
- Patient preferences regarding invasive procedures
- Historical adherence to follow-up appointments
- Healthcare system reliability in organizing referrals
- Risk assessment and resource allocation considerations
- Potential side effects of interventions
- Patient's social and economic circumstances
The Transformative Impact of Generative AI in Telehealth: Advancing Remote Healthcare Delivery. Read more here!
Methodology: A Rigorous Approach
The study utilized five de-identified patient cases to test clinical management reasoning across the three groups. Participants provided written responses detailing:
- What they would do in each case
- Why they chose that approach
- What factors they considered when making the decision
These responses were then evaluated against a rubric created by board-certified doctors that qualified what constituted appropriate medical judgment. This standardized approach allowed for objective comparison between groups.
Physicians with chatbot support spent approximately two minutes longer per case than those using conventional resources (519 seconds vs. 565 seconds), resulting in more thorough analyses. This additional time investment contributed to a lower risk of mild-to-moderate harm in treatment decisions (73.7% vs. 76.3%), though severe harm ratings remained similar between groups.

Evolution of AI in Medicine
This study builds upon earlier research published in JAMA Network Open in November 2024, which established that AI chatbots demonstrated higher accuracy than physicians in disease diagnosis.
According to a University of Virginia Health System study, chatbots had a median diagnostic accuracy exceeding 92%, outperforming unaided physicians.
The progression from diagnostic to management capabilities represents a significant advancement in AI in healthcare applications. The current study used GPT-4, a Large Language Model (LLM) that demonstrates remarkable reasoning abilities across multiple steps of clinical decision-making.
Previous research from Beth Israel Deaconess Medical Center, published in JAMA Internal Medicine, showed that ChatGPT-4 outperformed both attending physicians and residents in clinical reasoning assessments, achieving a median score of 10/10 compared to 9/10 for attending physicians and 8/10 for residents. However, that study also noted the chatbot had more instances of incorrect reasoning, highlighting the need for human oversight.
How Retrieval-Augmented Generation (RAG) Supports Healthcare AI Initiatives. Read more here!
Quality and Empathy: Unexpected Advantages
A separate study published in JAMA Internal Medicine in 2023 found that AI chatbot responses to patient questions were rated significantly higher in both quality and empathy compared to physicians' responses. When evaluated by healthcare professionals:
- AI chatbot responses were preferred over physician responses in 78.6% of evaluations
- The quality of information from chatbots was rated 3.6 times more often as "good" or "very good" compared to physicians
- AI chatbot responses were rated 9.8 times more often as "empathetic" or "very empathetic" than physician responses
These findings suggest that beyond clinical accuracy, AI chatbots may also enhance the patient experience through more thorough and empathetic communication—a critical aspect of healthcare delivery.
Implications for AI in Healthcare Practice
The findings suggest several potential benefits of integrating AI chatbots into clinical workflows:
- Enhanced decision quality: Physicians with AI assistance demonstrated more comprehensive assessment of patient cases, considering more factors in their decision-making
- Reduced cognitive load: AI chatbots can help physicians organize complex medical information and remind them of considerations they might otherwise overlook
- Improved safety: Lower rates of potential harm in decisions made with AI assistance suggest patient outcomes could improve
- Time efficiency: While physicians using chatbots spent slightly more time per case, the improved quality of decisions could reduce complications and follow-up visits
- Standardization of care: AI in medicine tools could help reduce variability in treatment approaches across different providers and settings
Dr. Jonathan Chen emphasizes that despite these promising results, "This doesn't mean patients should skip the doctor and go straight to chatbots. Don't do that. There's a lot of good information out there, but there's also bad information. The skill we all have to develop is discerning what's credible and what's not right. That's more important now than ever."
AI Meets Healthcare: How Asia-Pacific is Pioneering the Next Era of Medtech Innovation. Read more here!
Challenges and Limitations
Despite the positive outcomes, several challenges remain before widespread clinical implementation of AI in healthcare:
- Training needs: Physicians likely require specific training to effectively use Large Language Models as decision support tools
- Prompt design: The study suggests prompt engineering significantly impacts results, indicating healthcare organizations may need to develop standardized prompting strategies
- Integration into workflow: Adding AI in medicine tools must not disrupt clinical workflows or add administrative burden
- Trust calibration: Clinicians need to develop appropriate levels of trust in AI recommendations—neither over-relying nor dismissing them
- Ethical considerations: Questions about responsibility, liability, and informed consent remain unresolved
- Data privacy: Ensuring patient information remains protected when using LLM systems is paramount
The study did not investigate why physician-chatbot teams performed better—whether the AI chatbots provided novel insights or simply encouraged more methodical thinking remains unclear.
Dr. Stephanie Cabral, a researcher from Beth Israel Deaconess Medical Center, suggested that "AI could serve as a useful checkpoint to prevent oversight," but more research is needed on optimal implementation strategies.
Ongoing Research Initiatives
Research institutions including Stanford Medicine, Harvard University, the University of Minnesota, and the University of Virginia are continuing to explore how AI in medicine can best enhance patient care.
The establishment of a bi-coastal AI evaluation network called ARiSE (AI Research and Science Evaluation) signals growing interest in rigorously validating AI in healthcare applications.
According to Dr. Adam Rodman, director of AI Programs at Beth Israel Deaconess Medical Center, follow-up studies are already underway, moving into two subsequent phases:
- Investigating different types of AI chatbots, user interfaces, and physician education approaches to optimize performance
- Testing LLM systems with real-time patient data rather than archived cases to evaluate real-world effectiveness
These studies will include HIPAA-compliant, secure Large Language Models suitable for actual clinical environments, bringing research findings closer to practical implementation.
Thus,
The integration of AI chatbots into clinical practice represents a promising avenue for enhancing medical decision-making. Rather than replacing physician judgment, these tools appear to complement human expertise, potentially improving both the quality and safety of patient care.
As Dr. Chen suggests, this research challenges us to reconsider "what is a computer good at, what is a human good at," and how these capabilities can be optimally combined to benefit patients.
The complementary strengths of humans and AI in medicine suggest a future where collaboration, rather than competition, drives healthcare innovation. Physicians bring contextual understanding, empathy, and clinical experience, while Large Language Models contribute rapid information processing, pattern recognition, and standardization.
As healthcare systems continue to face increasing demands, electronic patient messages have increased 1.6-fold, contributing to physician burnout and stress. The thoughtful integration of AI in healthcare assistants may prove valuable in supporting physicians, reducing administrative burden, and ultimately improving the patient experience.
While we are still in the early stages of understanding how to best implement these technologies, the evidence increasingly suggests that the future of AI in medicine will involve human-AI partnerships rather than AI replacement of human clinicians.
Ready to enhance your healthcare practice with AI?
Makebot's healthcare-optimized LLM solutions can help your medical team make better clinical decisions. Our specialized MakeH system is already transforming patient care in major hospitals with 90% reservation efficiency.
Contact us today at b2b@makebot.ai to learn how our AI chatbot technology can integrate with your existing workflows and improve patient outcomes while reducing physician burnout.