Use Cases for Natural Language Processing in Healthcare
NLP in healthcare streamlines data, boosts decision-making, and enhances patient care efficiency.
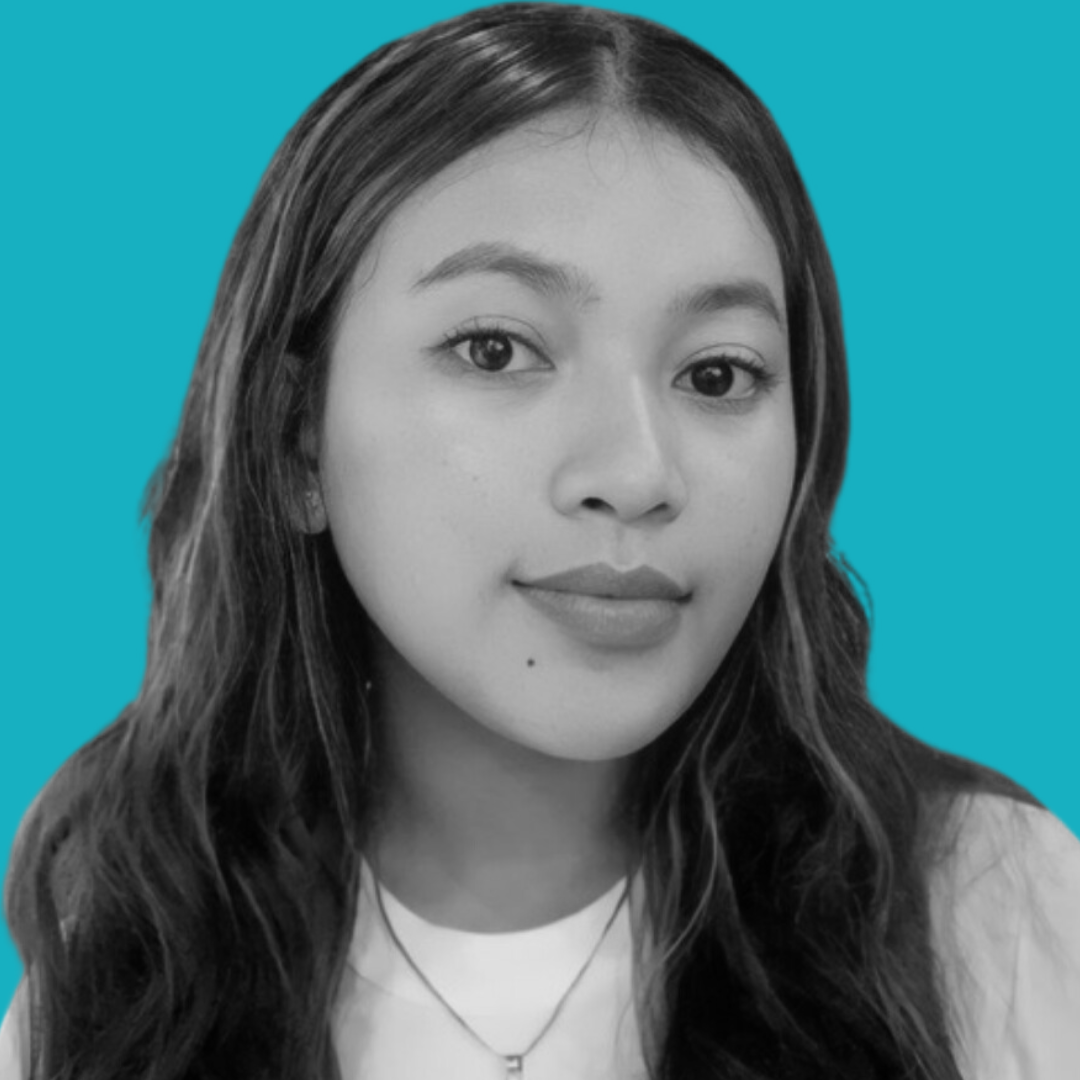
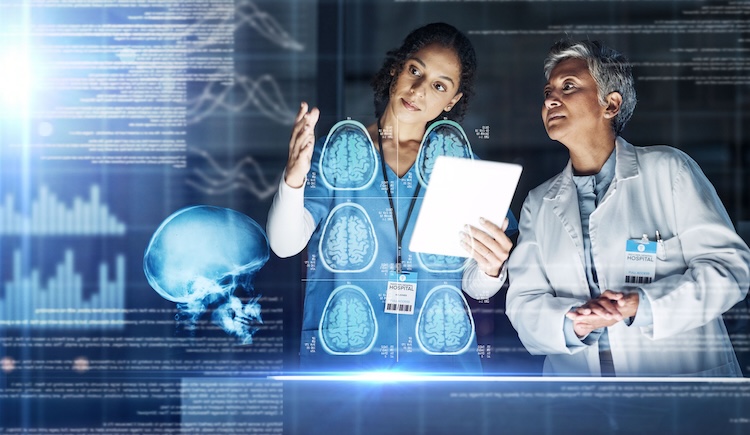
The healthcare industry generates vast amounts of unstructured data, with approximately 80% of all medical information existing in formats like clinical notes, medical records, research papers, and patient narratives.
Primary care physicians spend up to six hours daily on manual data entry, creating significant inefficiencies in healthcare delivery systems.
Natural Language Processing (NLP in healthcare) has emerged as a transformative technology that can address these challenges by enabling computers to understand, interpret, and generate human language from unstructured data.
The global market for NLP in healthcare and life sciences was valued at $4.9 billion in 2023 and is projected to grow at a compound annual growth rate (CAGR) of 34.7% through 2030. This rapid adoption reflects the technology's potential to revolutionize patient care, operational efficiency, and clinical research.
This article examines key use cases for Natural Language Processing in healthcare, demonstrating how this technology is reshaping the medical landscape through improved data accessibility, enhanced clinical decision-making, and streamlined administrative processes.
The Evolution from NLP to Generative AI Chatbots in 2025. Read more here!

Understanding NLP in Healthcare
Natural Language Processing represents a branch of artificial intelligence focused on the interaction between computers and human language.
In healthcare applications, NLP technologies process unstructured text and speech data to extract meaningful insights, patterns, and information that can support clinical practice and research.
Traditional data analysis tools often struggle with unstructured textual data, limiting healthcare organizations' ability to leverage the wealth of information contained in clinical notes, research literature, and patient communications.
NLP in healthcare addresses this gap by employing sophisticated algorithms to transform unstructured text into structured, analyzable data that can inform decision-making and improve patient outcomes.
10 Healthcare Challenges Solved by AI Chatbots and LLMs. Read more here!
Types of NLP Techniques in Healthcare
Several key NLP techniques are commonly applied in healthcare settings:
- Named Entity Recognition (NER): Identifies and categorizes key medical entities such as diseases, medications, procedures, and anatomical references in clinical text.
- Concept Unique Identifier (CUI) Extraction: Maps medical terms to standardized concept identifiers in systems like UMLS (Unified Medical Language System) to ensure consistent terminology.
- Sentiment Analysis: Evaluates emotional tone in patient communications and feedback to understand patient experiences.
- Topic Modeling: Uses approaches like Latent Dirichlet Allocation (LDA) to identify themes and patterns in large medical text collections.
- Relation Extraction: Identifies relationships between medical entities (e.g., drug-disease interactions, anatomical relations).
- Text Classification: Categorizes medical documents by purpose, content type, or clinical relevance.
- Medical Term Normalization: Standardizes variations in medical terminology, including abbreviations and acronyms.

Key Use Cases for NLP in Healthcare
1. Clinical Documentation Automation
Challenge: Clinical documentation is time-consuming, with physicians spending nearly 50% of their workday on documentation tasks. Manual data entry increases the risk of errors and reduces time available for direct patient care.
NLP Solution: Natural Language Processing technologies automate documentation processes by:
- Converting spoken narrative into structured text through speech recognition
- Extracting relevant clinical concepts from physician notes
- Automating the coding of diagnoses and procedures
- Identifying documentation gaps for regulatory compliance
Benefits:
- Reduces documentation time by up to 70%
- Improves accuracy of clinical documentation
- Enhances physician satisfaction by reducing administrative burden
- Increases time available for direct patient interaction
Real-World Application:
Solutions like M*Modal and Dragon Medical One leverage advanced speech recognition technology powered by NLP in healthcare to convert spoken language into structured information directly within electronic health record (EHR) systems. These technologies can learn from physician preferences, ensuring outputs align with medical standards and clinical workflows.
2. Medical Record Analysis and Risk Prediction
Challenge: Traditional methods for analyzing patient records rely on structured data fields, missing valuable information contained in unstructured clinical notes. This limits the ability to identify risk factors and predict adverse events.
NLP Solution: Natural Language Processing technologies analyze unstructured clinical text to:
- Extract risk factors not captured in structured data
- Identify patterns predictive of disease progression
- Recognize early warning signs of clinical deterioration
- Enhance population health management efforts
Benefits:
- Provides more comprehensive patient risk profiles
- Enables earlier intervention for high-risk patients
- Improves accuracy of predictive models by incorporating textual data
- Supports more personalized care management strategies
Real-World Application:
Research has demonstrated that NLP in healthcare can identify psychosocial factors (such as isolation, housing instability, financial insecurity, and stress) from chart notes and predict their impact on one-year hospitalization rates.
Models incorporating these NLP-extracted factors showed significantly improved predictive performance compared to models using structured data alone.
A study on ICU mortality prediction found that NLP-enhanced models achieved an AUC of 0.922, compared to 0.831 for baseline models without NLP-derived features, demonstrating the substantial improvement gained by incorporating information from clinical text.
3. Adverse Drug Event Detection
Challenge: Adverse drug events (ADEs) are often documented in clinical notes rather than structured fields, making them difficult to identify systematically. Traditional pharmacovigilance methods may miss important signals in unstructured text.
NLP Solution: Natural Language Processing systems can:
- Automatically detect mentions of adverse drug reactions in clinical notes
- Identify drug-symptom relationships indicating potential ADEs
- Monitor social media and patient forums for emerging adverse event signals
- Support regulatory reporting and post-marketing surveillance
Benefits:
- Enables earlier detection of potential drug safety issues
- Improves comprehensive monitoring of medication effects
- Reduces manual review burden for pharmacovigilance teams
- Enhances patient safety through more complete ADE detection
Real-World Application:
NLP in healthcare applications can analyze patient reviews, social media posts, and clinical documentation to detect relations between drugs and adverse reactions.
For example, a study analyzing Twitter data identified consistent coverage of adverse events following immunization (AEFI) for COVID-19 vaccines compared to governmental sources, supporting social media analysis as a complementary approach to conventional pharmacovigilance.
4. Clinical Trial Matching and Management
Challenge: Identifying eligible patients for clinical trials through manual chart review is labor-intensive and often inefficient. Traditional methods may miss potential participants or include ineligible ones, affecting trial recruitment and outcomes.
NLP Solution: Natural Language Processing technologies facilitate clinical trial processes by:
- Automating screening of patient records against trial eligibility criteria
- Extracting relevant clinical information from unstructured notes
- Identifying potential trial candidates based on comprehensive clinical profiles
- Monitoring protocol adherence and outcomes documentation
Benefits:
- Accelerates patient recruitment for clinical trials
- Improves matching accuracy between patients and appropriate trials
- Reduces administrative burden associated with trial management
- Enhances diversity in clinical trial participation through broader screening
Real-World Application:
NLP systems have been developed to automate the trial matching process, reducing manual effort and enhancing efficiency in identifying suitable candidates. Companies like IBM Watson Health and Inspirata are leveraging Natural Language Processing for oncology trials, improving the accuracy and speed of patient matching.
5. Medical Image Analysis and Reporting
Challenge: Radiological and pathological reports contain valuable information in unstructured text format that is difficult to analyze systematically. This limits the ability to extract structured insights from imaging studies.
NLP Solution: Natural Language Processing can enhance medical imaging workflows by:
- Extracting key findings from radiology and pathology reports
- Converting unstructured imaging reports into structured data
- Automating the categorization of findings by severity and urgency
- Supporting quality assurance and follow-up recommendation tracking
Benefits:
- Enables systematic analysis of radiological and pathological findings
- Improves follow-up adherence for incidental findings
- Enhances research capabilities through structured analysis of imaging reports
- Supports quality improvement initiatives in diagnostic imaging
Real-World Application:
NLP in healthcare has been used to automatically infer BI-RADS final assessment categories from narrative mammography reports with high accuracy.
A study by Banerjee et al. demonstrated that NLP classifiers could recognize contextual information that enabled accurate determination of BI-RADS categorization from narrative reports.
Additionally, NLP has been successfully used in "identifying critical findings, categorizing oncologic responses, finding follow-up recommendations, identifying pulmonary emboli, detecting complications of stroke, and classifying epilepsy brain MRIs," demonstrating its broad utility in radiology workflows.
6. Medical Literature Analysis and Knowledge Extraction
Challenge: The volume of medical literature is growing exponentially, making it impossible for clinicians to stay current with relevant research. Manual literature reviews are time-consuming and may miss important findings.
NLP Solution: Natural Language Processing technologies can revolutionize knowledge management by:
- Automatically analyzing research papers and clinical guidelines
- Extracting key findings and recommendations from medical literature
- Identifying emerging trends and experimental treatments
- Supporting evidence-based practice through literature summarization
Benefits:
- Enables more comprehensive literature reviews in less time
- Keeps clinicians updated on latest research relevant to their patients
- Supports evidence-based clinical decision-making
- Accelerates translational research by connecting clinical needs with research findings
Real-World Application:
A study by Karabacak et al. used NLP in healthcare to analyze topics and trends in neurosurgical research. The analysis identified both prominent and underrepresented topics in the current decade, demonstrating how NLP techniques can help understand research landscapes and guide future investigations.
Large Language Models (LLMs) and other advanced NLP in healthcare technologies can process vast amounts of medical literature, extract key insights, and present them in digestible formats.
This capability is particularly valuable for systematic reviews, where Natural Language Processing can significantly reduce the time required for initial screening and data extraction.
7. Patient Experience and Sentiment Analysis
Challenge: Understanding patient experiences and satisfaction traditionally relies on structured surveys with limited participation. Valuable patient feedback often exists in unstructured formats across various platforms.
NLP Solution: Natural Language Processing enables comprehensive analysis of patient sentiment by:
- Analyzing patient reviews and feedback across multiple channels
- Identifying emerging patient concerns and satisfaction drivers
- Extracting themes from patient narratives about their healthcare experiences
- Tracking sentiment trends over time to measure improvement initiatives
Benefits:
- Provides deeper insights into patient experiences beyond survey data
- Enables proactive identification of service issues affecting patient satisfaction
- Supports targeted improvement initiatives based on patient feedback
- Enhances understanding of patient perspectives on care quality
Real-World Application:
NLP in healthcare applications can analyze sentiment in patient reviews and feedback, identifying both positive and negative patterns. Healthcare organizations can leverage these insights to address service gaps, improve communication, and enhance overall patient experience.
8. Public Health Surveillance and Epidemiology
Challenge: Traditional public health surveillance systems often rely on structured reporting mechanisms with inherent delays. Valuable early signals of disease outbreaks may exist in unstructured data sources.
NLP Solution: Natural Language Processing technologies can enhance public health monitoring by:
- Analyzing social media and web content for disease outbreak signals
- Processing clinical notes to identify unusual symptom patterns
- Monitoring news sources for emerging public health threats
- Extracting epidemiological information from scientific literature
Benefits:
- Enables earlier detection of potential disease outbreaks
- Provides real-time insights into public health trends
- Supports more timely and targeted public health interventions
- Enhances understanding of disease patterns and risk factors
Real-World Application:
During the COVID-19 pandemic, Natural Language Processing was employed to analyze social media data to evaluate public sentiment regarding vaccines and track symptom reporting.
A study funded by the Japan Science and Technology Agency used NLP to analyze 144,101 tweets related to COVID-19 vaccination in Japan, helping professionals identify relationships between public views and infection rates, deaths, and vaccination levels.
The LDA model identified approximately 85% of tweets expressing neutral opinions, with negative opinions outweighing positive ones in the remaining 15%.
Another study compared public responses to COVID-19 and monkeypox on Twitter in Germany, finding distinct differences in public attitudes toward each health crisis. Results showed minimal regard for precautionary actions during monkeypox, while COVID-19 discussions focused more on protective measures as death rates increased.
These findings suggest that NLP tools for monitoring public sentiment could be leveraged to promote various health policies more effectively.
9. Computer-Assisted Coding and Revenue Cycle Management
Challenge: Medical coding is complex, time-consuming, and prone to errors. Inaccurate coding can lead to claim denials, revenue loss, and compliance risks.
NLP Solution: Natural Language Processing supports medical coding by:
- Automatically extracting billable diagnoses and procedures from clinical notes
- Suggesting appropriate billing codes based on documentation
- Identifying documentation gaps that could affect reimbursement
- Detecting potential coding errors and compliance issues
Benefits:
- Reduces coding time and effort by up to 50%
- Improves coding accuracy and consistency
- Accelerates billing cycles and reduces claim denials
- Enhances compliance with coding regulations and requirements
Real-World Application:
Computer-assisted coding powered by NLP in healthcare has become one of the most widely adopted applications, though with adoption rates of only around 30%. These systems capture data on procedures and treatments to identify appropriate billing codes, maximizing revenue while ensuring compliance.
10. Social Determinants of Health Identification
Challenge: Social determinants of health (SDOH), such as housing, food access, and income, significantly influence health outcomes but are often inadequately captured in standard clinical assessments.
NLP Solution: NLP in healthcare can analyze unstructured clinical notes to:
- Identify mentions of social factors affecting patient health
- Flag issues like housing instability or food insecurity
- Enable more comprehensive understanding of patient circumstances
- Support coordinated care that addresses root causes of health issues
Benefits:
- Provides a more holistic view of patient health
- Enables care plans tailored to individual social circumstances
- Supports improved management of complex health conditions
- Facilitates coordination with social services and community resources
Real-World Application:
NLP technologies analyze unstructured data in clinical notes to identify social factors that may not be systematically recorded elsewhere. This information helps healthcare professionals develop comprehensive care plans that address both medical and social needs, ultimately improving health outcomes.
Market Growth Transforming Healthcare with AI Chatbots, GenAI, and LLMs. Read more here!
Advanced NLP Technologies in Healthcare
The Role of Large Language Models
Large Language Models (LLMs) represent a significant advancement in Natural Language Processing, with profound implications for healthcare applications. These models, trained on vast text corpora, can understand context, generate human-like text, and perform complex language tasks with unprecedented accuracy.
In healthcare, LLMs are being leveraged for various applications, including:
- Clinical Decision Support: LLMs can analyze patient records, relevant literature, and clinical guidelines to provide evidence-based recommendations to clinicians.
- Medical Documentation: Advanced LLMs can generate comprehensive clinical notes from physician-patient conversations, reducing documentation burden.
- Patient Communication: LLMs can simplify complex medical information for patients, enhancing understanding and engagement in care.
- Medical Education: These models can create realistic clinical scenarios for training, providing personalized educational experiences for medical students and professionals.
A study published in the Yale Journal of Biology and Medicine highlighted how LLMs could be used to simplify imaging reports for patients in response to the 21st Century Cures Act, which expanded patient access to their electronic health information.
Medical reports are often complex and filled with technical terminology that patients struggle to understand, but LLMs can translate these into more accessible language without creating additional work for radiologists.
The integration of LLMs in healthcare workflows represents a significant evolution in NLP in healthcare, enabling more sophisticated analysis and generation of medical text.
LLMs in Healthcare: September 2024's Medical Breakthroughs. Read more here!
Generative AI in Healthcare Applications
Generative AI technologies are transforming healthcare by creating new content based on patterns learned from existing data. In medical contexts, Generative AI applications include:
- Report Generation: Automatically creating structured clinical documents from unstructured inputs like dictation or conversations.
- Patient Education Materials: Generating personalized health information tailored to individual patient needs and literacy levels.
- Clinical Trial Protocol Development: Creating draft protocols based on target population characteristics and treatment mechanisms.
- Synthetic Data Generation: Producing realistic but de-identified patient data for research and algorithm training purposes.
These Generative AI applications demonstrate how advanced NLP in healthcare is moving beyond analysis to content creation, supporting various clinical and operational needs.
90% of Healthcare Executives See Positive ROI from GenAI Investments. Read more here!
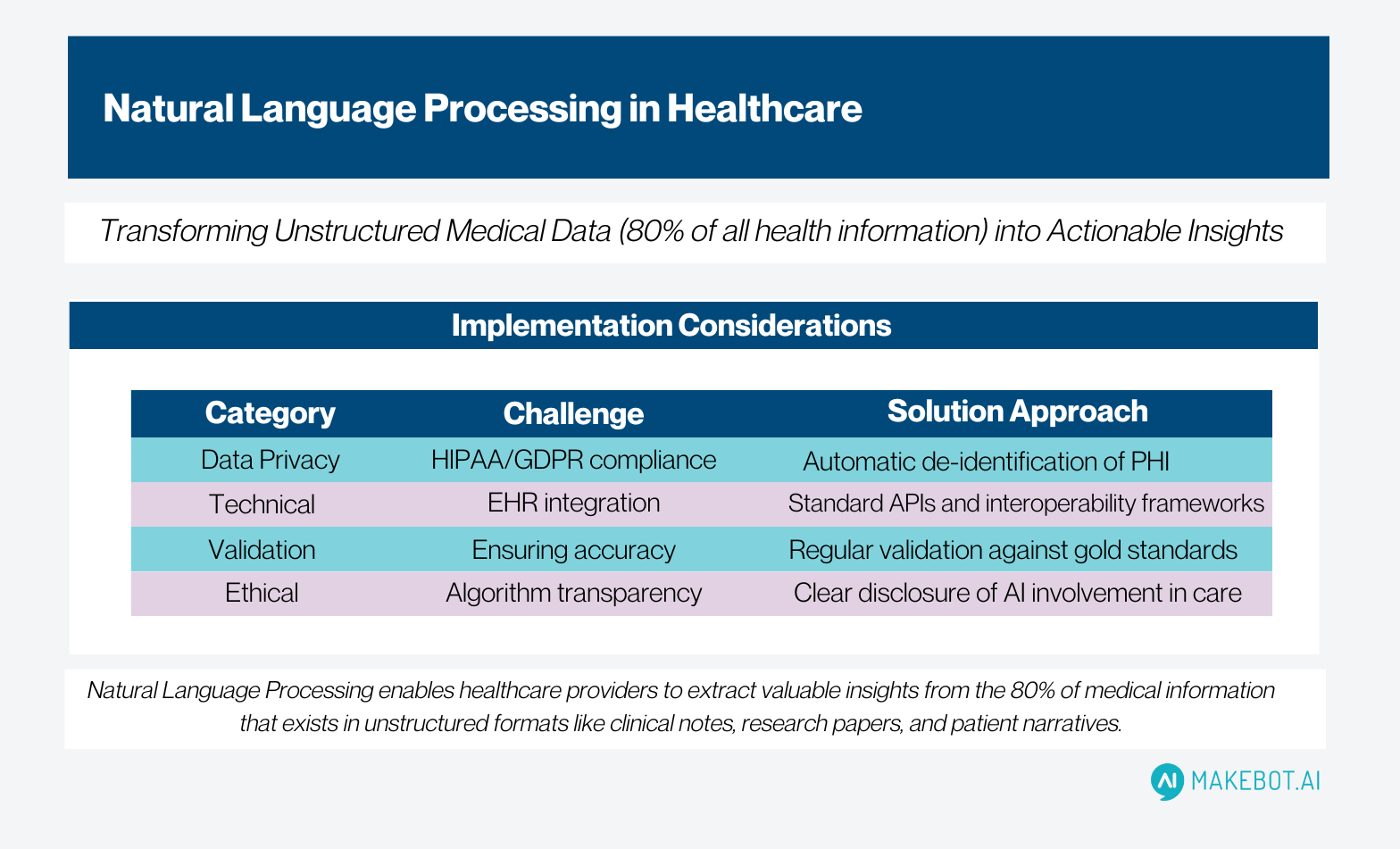
Implementation Considerations and Challenges
Data Privacy and Security
Implementing NLP in healthcare requires careful attention to data privacy regulations like HIPAA and GDPR. Considerations include:
- De-identification of protected health information before processing
- Secure storage and transmission of data containing patient information
- Transparent consent processes for NLP applications using patient data
- Audit trails for access to and use of sensitive information
Several studies highlight the importance of de-identification in healthcare NLP applications. Advanced NLP tools can automatically identify and remove personal and clinical information from medical documents, including patient names, dates, doctor information, hospital names, IDs, medical record numbers, and other protected health information (PHI).
These systems can process documents in multiple languages and formats, including structured datasets, PDF documents, and DICOM images.
Technical Integration
Successful integration of Natural Language Processing technologies into healthcare workflows requires:
- Compatibility with existing electronic health record systems
- Scalable infrastructure to handle large volumes of textual data
- Well-defined APIs for interoperability with other healthcare applications
- Ongoing maintenance and updates to accommodate evolving clinical terminology
Model Validation and Accuracy
Ensuring the reliability of NLP in healthcare applications involves:
- Rigorous validation against gold-standard manual reviews
- Regular performance monitoring and retraining to maintain accuracy
- Domain-specific optimization for medical terminology and contexts
- Transparent reporting of model limitations and performance metrics
A study on serum protein electrophoresis reports in the Veterans Affairs Health Care System demonstrated that NLP systems could achieve extremely high accuracy (AUC of 0.997) in identifying monoclonal gammopathy, comparable to manual review (AUC of 0.99).
This suggests that binary classification of clinical documents can achieve excellent performance with well-designed NLP systems.
Ethical Considerations
Responsible implementation of NLP in healthcare must address:
- Potential biases in training data that could affect equitable care delivery
- Appropriate roles for automation versus human judgment in clinical contexts
- Clear disclosure of AI/NLP involvement in patient care processes
- Accountability frameworks for decisions influenced by NLP outputs
A study emphasizes that patients should be informed that algorithms can be imperfect or wrong, algorithm data should be considered highly sensitive, algorithm data might recommend actions that are not immediately apparent, and algorithms might prompt unnecessary intervention from providers. This transparency is essential for maintaining trust and ensuring ethical use of NLP technologies in healthcare.
Future Directions
The evolution of Natural Language Processing in healthcare is likely to accelerate, driven by advances in Large Language Models, Generative AI, and multimodal approaches that combine text analysis with other data types. Emerging trends include:
- Multimodal NLP: Integration of text analysis with medical imaging, genomic data, and physiological signals for more comprehensive insights.
- Ambient Clinical Intelligence: Systems that passively listen to clinical encounters, automatically documenting and providing real-time decision support.
- Personalized NLP: Models tailored to individual clinician communication styles and specialty-specific terminology.
- Federated Learning: NLP models trained across institutions without sharing sensitive data, enabling broader and more diverse training while maintaining privacy.
- Explainable NLP: Increased transparency in how NLP systems reach conclusions, building trust and supporting clinical adoption.
Audio-based NLP applications show particular promise for enhancing data collection and analysis in healthcare. A study proposed examining audio recordings of patient visits to capture comprehensive symptom data for COVID-19, noting that this approach could compensate for limitations in electronic health records, where symptoms might not be accurately transcribed.
The integration of audio data from clinical encounters, telehealth appointments, and voice-activated systems could improve diagnostic accuracy by capturing a broader range of symptoms and patient expressions.
Thus,
Natural Language Processing has emerged as a transformative technology in healthcare, unlocking the value of unstructured data that previously remained inaccessible to systematic analysis.
From clinical documentation and decision support to research acceleration and public health surveillance, NLP in healthcare applications are addressing critical challenges across the healthcare ecosystem.
As Large Language Models and Generative AI continue to advance, the capabilities and impact of Natural Language Processing in healthcare will likely expand further. However, successful implementation requires careful attention to technical, ethical, and practical considerations to ensure these powerful technologies enhance rather than complicate healthcare delivery.
Healthcare organizations embarking on NLP in healthcare initiatives should adopt a strategic approach, identifying high-value use cases with measurable outcomes, ensuring appropriate infrastructure and expertise, and establishing robust governance frameworks.
With thoughtful implementation, Natural Language Processing can help transform healthcare delivery, making it more efficient, effective, and equitable.
Transform Healthcare Operations with AI-Powered Solutions
As NLP revolutionizes healthcare with 80% of medical data existing in unstructured formats, Makebot's expertise in hybrid RAG technology offers the perfect solution for medical organizations looking to unlock this valuable information.
Ready to minimize documentation time by up to 70% and improve patient care?
Our specialized MakeH solution has already achieved a 90% reservation rate in leading hospitals. Contact our healthcare AI experts today at b2b@makebot.ai to discover how our verified chatbot solutions can transform your medical practice.