LLMs in Healthcare: September 2024's Medical Breakthroughs
LLMs in Healthcare: September 2024's medical breakthroughs.
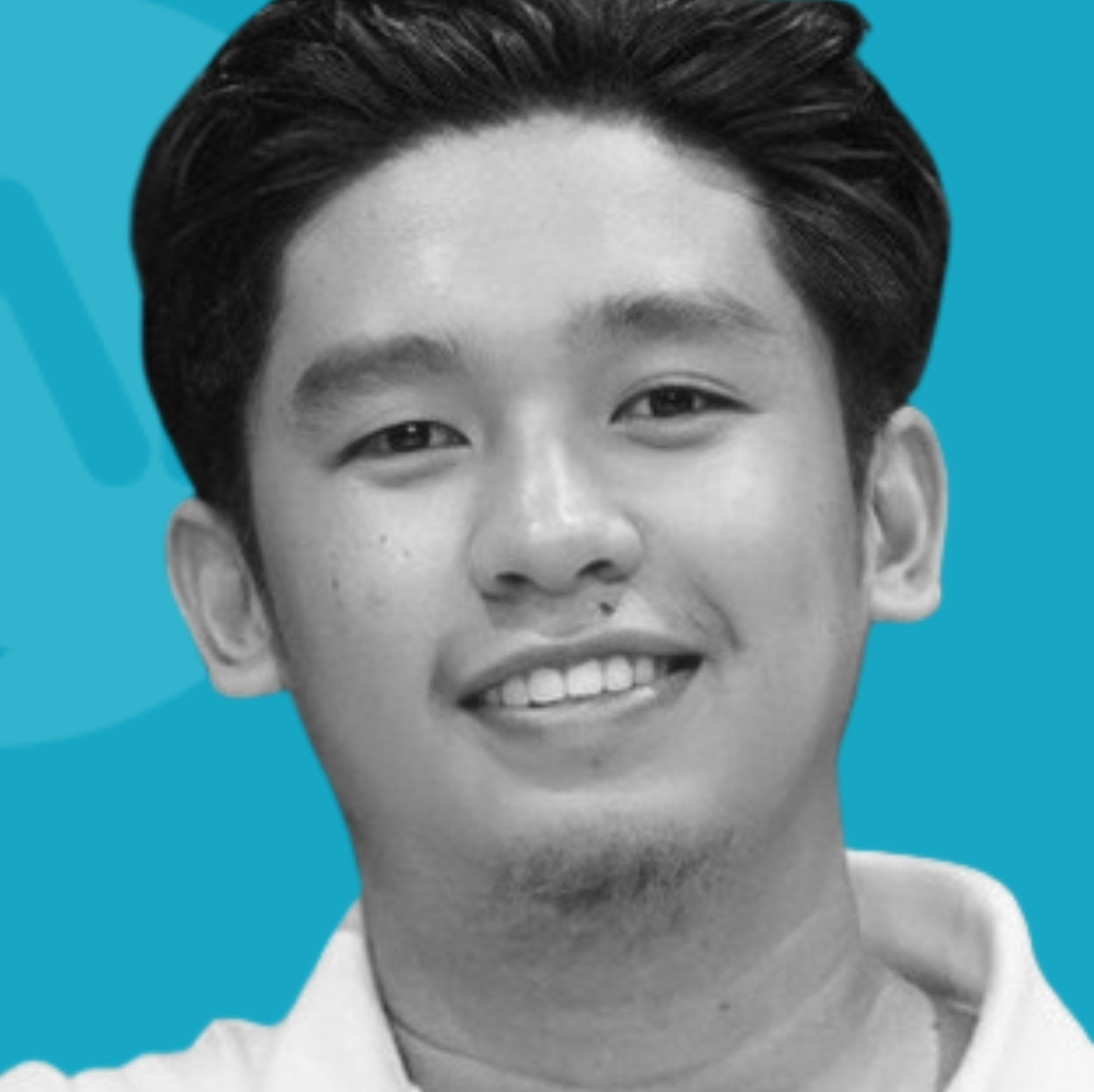
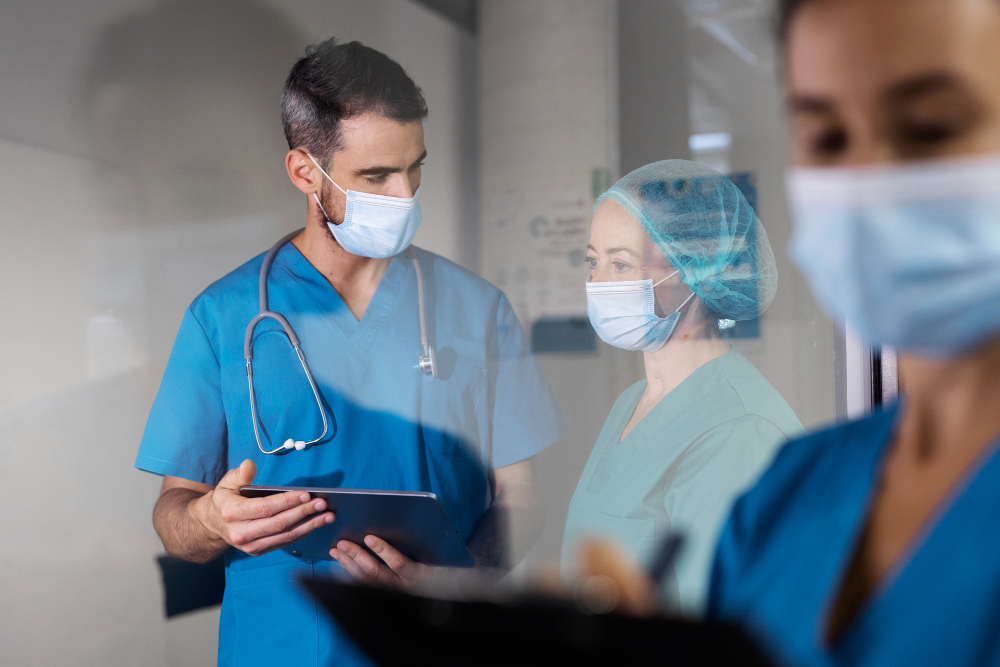
How are large language models (LLMs) shaping the future of healthcare?
Can AI-powered diagnostics revolutionize the way we detect and treat diseases?
September 2024 delivered groundbreaking insights regarding large language models in healthcare, as significant investments, cutting-edge research, and new performance data reveal the accelerating impact of LLMs in the medical field.
With open-source models closing the gap on proprietary alternatives, and AI diagnostics improving accuracy by over 16%, it’s clear that LLMs are driving a healthcare revolution.
But how far can this technology go, and what challenges must still be overcome? Let’s dive into the data and explore.

A Surge in Investment: LLMs Gain Traction in Healthcare
Large Language Models in the healthcare industry have become a cornerstone, with substantial financial backing leading the way.
In September 2024, Hippocratic AI, a company specializing in safety-focused LLMs for healthcare, received an additional $17 million investment from NVentures. This brings their total funding to $137 million.
The investment supports the scaling of real-time, long-context conversational AI capabilities, using NVIDIA NIM microservices to improve patient-clinician interactions.
The financial surge mirrors the broader market trends. The global artificial intelligence (AI) in healthcare market, valued at $16.3 billion in 2022, is projected to reach $173.55 billion by 2029, growing at an extraordinary compound annual growth rate (CAGR) of 40.2%.
Key Points:
- Total investment in Hippocratic AI: $137 million
- Global healthcare AI market growth: From $16.3 billion in 2022 to $173.55 billion by 2029 (CAGR: 40.2%)
- Number of healthcare certifications targeted by Hippocratic AI’s LLM: 114
With increasing investments, the focus now shifts to closing the performance gap between open-source and proprietary LLMs, crucial for the future of accessible AI-driven healthcare.
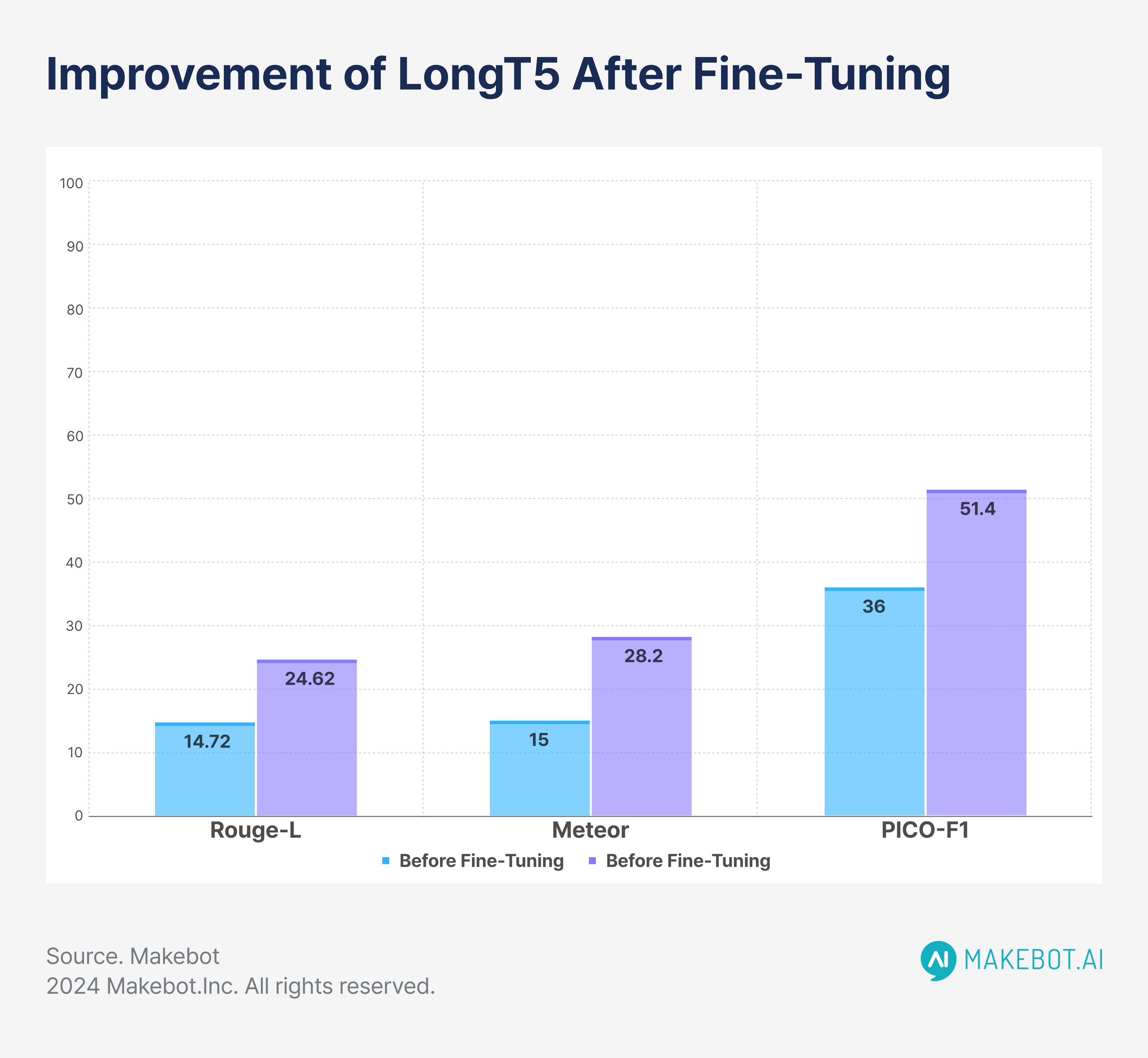
Closing the Performance Gap: Open-Source vs. Proprietary LLMs
Proprietary LLMs such as GPT-3.5 and GPT-4 have set benchmarks in healthcare, but recent studies show that fine-tuned open-source models are quickly catching up.
More on GPT here: Is Chatgpt Generative AI? (How to Make the Best of it)
In a landmark study conducted in September 2024, fine-tuned LongT5, one of the most widely used open-source LLMs, dramatically improved its performance. The ROUGE-L score rose from 14.72 to 24.61, and METEOR scores surged from 15.06 to 28.27.
This allowed LongT5 to match GPT-3.5’s performance in some areas, such as medical summarization tasks.
Interestingly, fine-tuning smaller models can sometimes yield better results. In the same study, LongT5-base, with fewer parameters, outperformed the zero-shot LongT5-xl by 38.81% in PICO-F1 score.
Key Points:
- ROUGE-L improvement: 14.72 to 24.61
- METEOR improvement: 15.06 to 28.27
- PICO-F1 improvement: 36.05 to 51.43
- Number of systematic reviews fine-tuned on MedReview: 8,161 pairs
As open-source models become more competitive, transparency and flexibility in healthcare AI become more attainable.
However, these performance gains reveal a new challenge: ensuring that Large Language Models in healthcare can withstand real-world clinical complexities, as highlighted by the MedFuzz robustness tests.
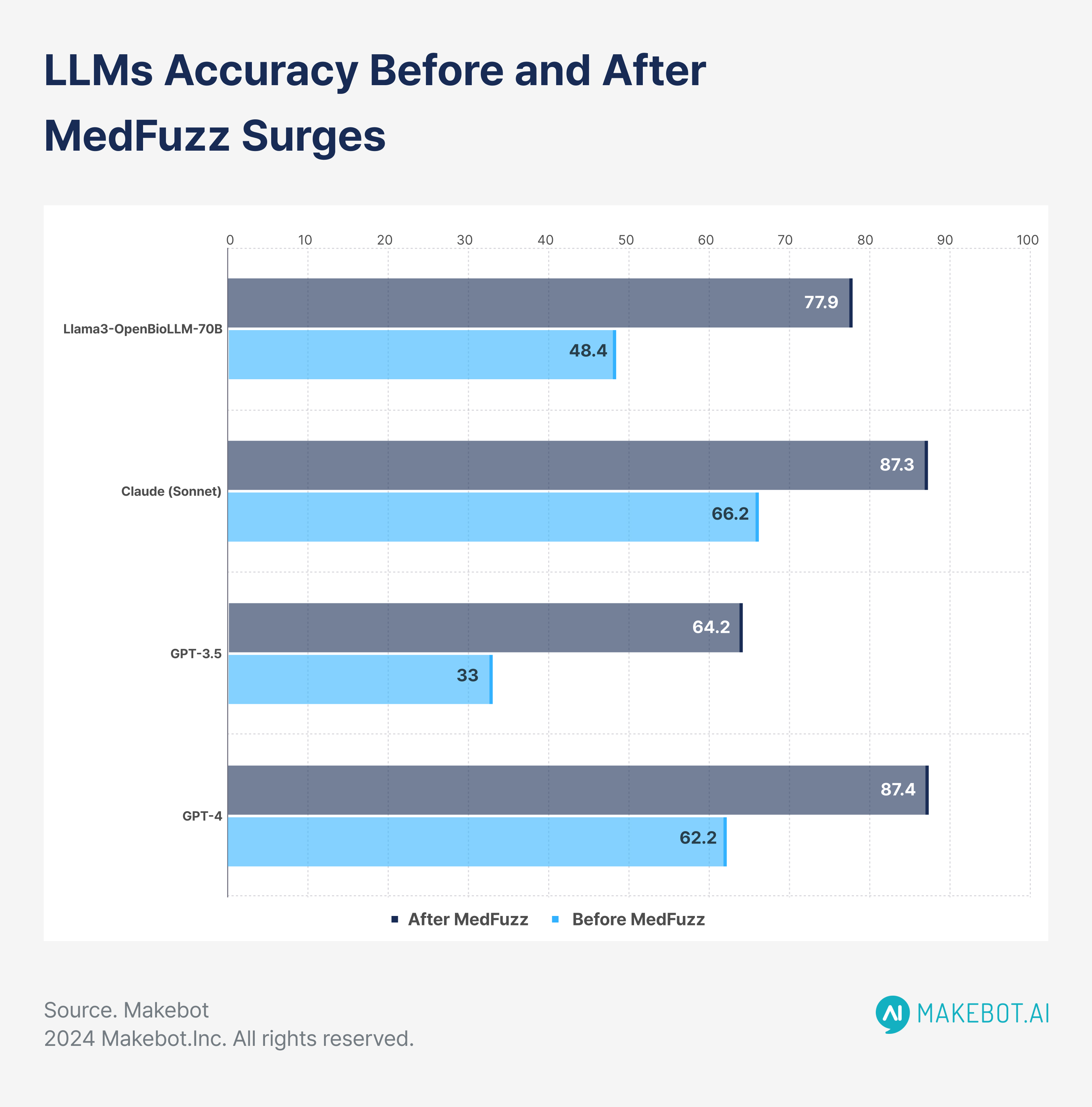
Robustness of LLMs: MedFuzz Exposes Weaknesses
While Large Language Models in the healthcare have showcased impressive results on medical benchmarks, real-world applications pose unique challenges.
MedFuzz, a tool developed by Microsoft Research, demonstrated that LLM performance drops significantly when subjected to more complex, real-world clinical scenarios. After repeated MedFuzz attacks, GPT-4's accuracy on medical benchmarks fell from 87.4% to 62.2%.
You may Read also: Things to Avoid when Prompting AI to Create Perfect Results
These findings stress the importance of improving LLMs' ability to handle nuanced clinical data, going beyond simplified benchmarks like MedQA.
The MedFuzz experiment highlighted vulnerabilities across various LLMs:
- GPT-3.5: From 64.2% to 33% accuracy after MedFuzz surges
- Claude (Sonnet): From 87.3% to 66.2% accuracy
- Llama3-OpenBioLLM-70B: From 77.9% to 48.4% accuracy
Key Points:
- GPT-4 accuracy drop after MedFuzz: From 87.4% to 62.2%
- GPT-3.5 accuracy drop after MedFuzz: From 64.2% to 33%
- MedFuzz testing scope: 378 simulated cases with 7 different LLMs
These robustness challenges emphasize the need for ongoing fine-tuning, leading directly into the benefits of LLM and CAD system integrations for improving diagnostic accuracy.
Interactive Medical Diagnosis: LLMs Meet CAD Systems
The combination of LLMs with computer-aided diagnosis (CAD) systems has opened new possibilities for interactive medical diagnostics.
More AI in Healthcare Industry on: Doctor AI: The Rise of Generative AI in Healthcare
In September 2024, researchers introduced a framework that integrates LLMs with CAD networks to generate more accurate medical diagnoses.
In a trial focused on chest X-rays, the system, powered by ChatGPT, improved diagnostic accuracy by 16.42% compared to traditional CAD systems.
The CAD-LLM system generated reports that outperformed human radiologists in several categories, with the F1 score improving by 15 percentage points when compared to baseline CAD models.
This breakthrough not only improved diagnostic quality but also created patient-friendly, interactive reports that simplify complex medical terms for non-experts.
Key Points:
- Diagnostic accuracy improvement: 16.42%
- F1 score improvement: 15 percentage points
- Number of cases tested: 300 chest X-rays
This success in enhancing diagnostics brings us to the future outlook, where LLMs are poised to become indispensable tools across multiple healthcare applications.
The Healthcare Revolution is Powered by LLMs
The data from September 2024 paints a clear picture: Large Language Models in healthcare are not just making waves—they're transforming the entire industry.
From Hippocratic AI’s $137 million investment to the 16.42% diagnostic accuracy improvement with CAD-LLM integration, the numbers tell a compelling story of rapid growth, innovation, and potential.
While challenges like real-world robustness exposed by MedFuzz remain, the strides made by fine-tuned open-source models, such as LongT5, show that we are closing the gap between proprietary models and more accessible alternatives.
As the global healthcare AI market is set to grow from $16.3 billion in 2022 to $173.55 billion by 2029, the future of healthcare is undoubtedly intertwined with the power of LLMs.
With continued investments, advancements in AI-driven diagnostics, and a focus on overcoming real-world complexities, LLMs are set to revolutionize patient care and healthcare systems around the globe.
We’re just at the beginning of an AI-driven transformation that will redefine how we approach healthcare—and the numbers confirm it.