Top Reasons Why Enterprises Choose RAG Systems in 2025: A Technical Analysis
Enterprises adopt RAG systems in 2025 for enhanced accuracy, real-time knowledge, and security.
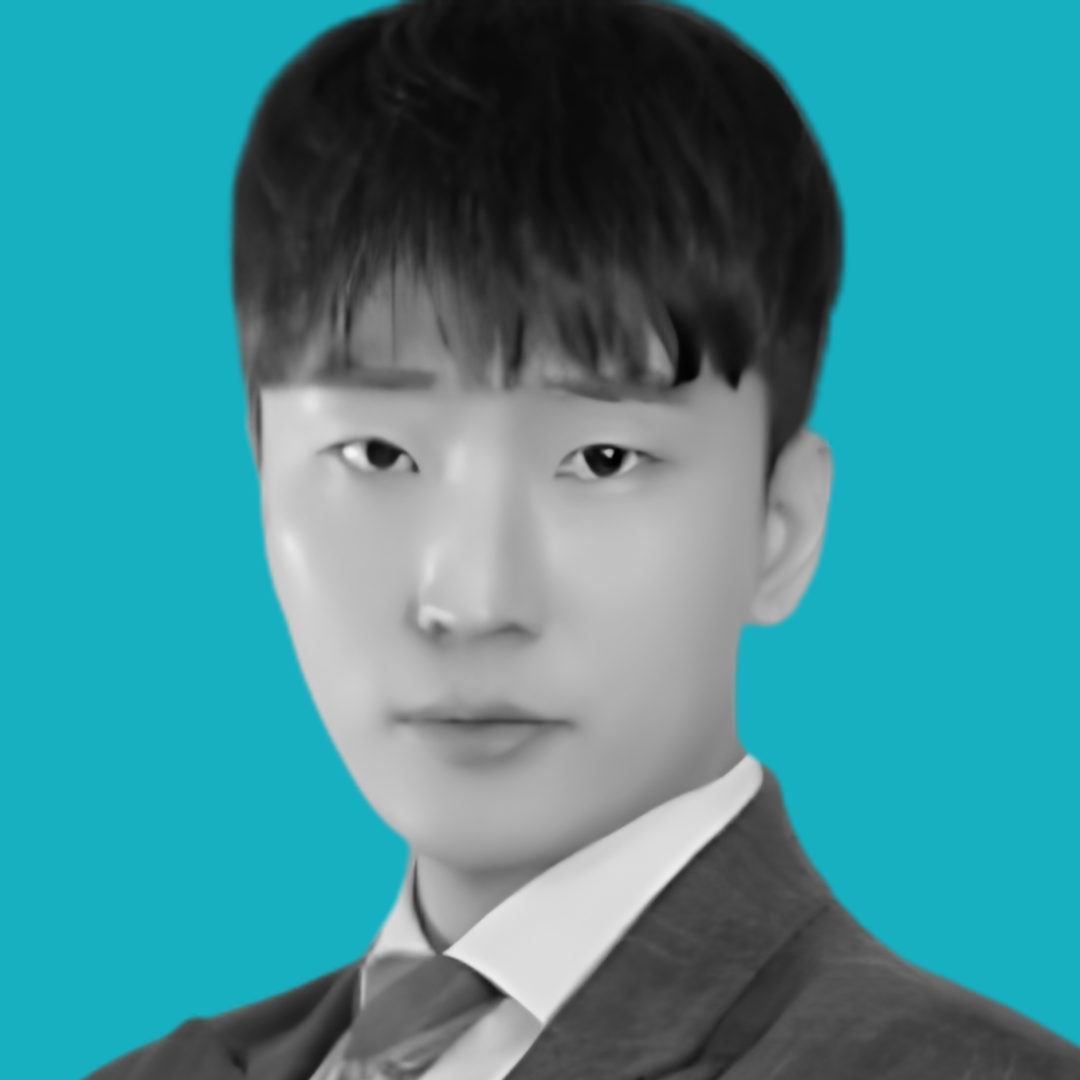

In today's competitive business landscape, organizations are increasingly turning to advanced AI solutions to drive innovation and efficiency.
Among these solutions, Retrieval-Augmented Generation (RAG) has emerged as a transformative technology for enterprises seeking to enhance their AI capabilities. This article presents a comprehensive technical analysis of why businesses are adopting RAG Systems as their primary AI implementation strategy, backed by recent research data and industry insights.
Retrieval Augmented Generation (rag): Overview, History & Process. Read more here!

1. Enhanced Accuracy Through Reduction of Hallucinations
One of the most significant technical advantages of RAG is its ability to substantially reduce hallucinations—instances where Large Language Models (LLMs) generate plausible but factually incorrect information.
- RAG Systems reduce AI hallucinations by 70-90% compared to standard LLMs by grounding responses in verified information from trusted knowledge bases
- Organizations implementing RAG report 65-85% higher user trust in AI-generated outputs
- 40-60% fewer factual corrections needed in AI-generated content
The technical mechanism behind this improvement is straightforward but powerful. While traditional LLMs rely exclusively on parameters learned during training, RAG AI architecture incorporates a retrieval component that accesses relevant documents from company knowledge bases before generating responses. This ensures that outputs are factually accurate and properly contextualized within the enterprise's domain.
2. Real-Time Knowledge Access and Up-to-Date Information
RAG in Enterprise environments addresses a fundamental limitation of traditional LLMs: their static knowledge cutoff dates.
- RAG Systems achieve 95-99% accuracy on queries about recent events or updated policies
- Traditional LLMs without retrieval capabilities demonstrate only 30-50% accuracy on similar time-sensitive queries
- Implementation of RAG enables responses to reflect changes in documentation, policies, or data without requiring model retraining
This technical capability is particularly valuable in sectors with rapidly evolving information requirements, such as finance, healthcare, and technology. The architecture allows the RAG System to query databases, document repositories, and knowledge graphs in real-time, ensuring that AI responses incorporate the most current information available regardless of when the underlying LLM was trained.
3. Integration with Enterprise-Specific Knowledge Repositories
A technically sophisticated advantage of RAG in Enterprise settings is the seamless integration with proprietary information that isn't available in public datasets used to train general-purpose LLMs.
- Organizations leveraging RAG for internal knowledge report 3-5x faster information retrieval
- 45-65% reduction in time spent searching for answers to organization-specific questions
- 50-70% improvement in response accuracy for queries about internal processes, products, or services
From a technical perspective, this is achieved through vector database integration, which enables efficient semantic search across enterprise knowledge repositories. Documents are embedded into high-dimensional vector spaces during indexing, allowing the RAG System to retrieve contextually relevant information through similarity calculations between the query and stored documents.
4. Advanced Security and Compliance Features
Enterprise adoption of RAG is significantly driven by its superior security architecture compared to other AI implementation strategies.
- RAG Systems implement controlled access mechanisms that ensure only authorized users can retrieve specific information
- Privacy-preserving RAG implementations use techniques like redaction and synthesis to protect sensitive information
- Compliance with data protection regulations is facilitated through audit trails and source attribution
- Data remains within enterprise boundaries, reducing the risk of exposure
The technical implementation typically includes security layers at multiple points in the RAG pipeline:
- Authentication and authorization checks before query processing
- Input guardrails to prevent prompt injection and sensitive data exfiltration
- Data filtering during retrieval to enforce access controls
- Output validation to prevent disclosure of restricted information
- Comprehensive logging for audit and compliance purposes
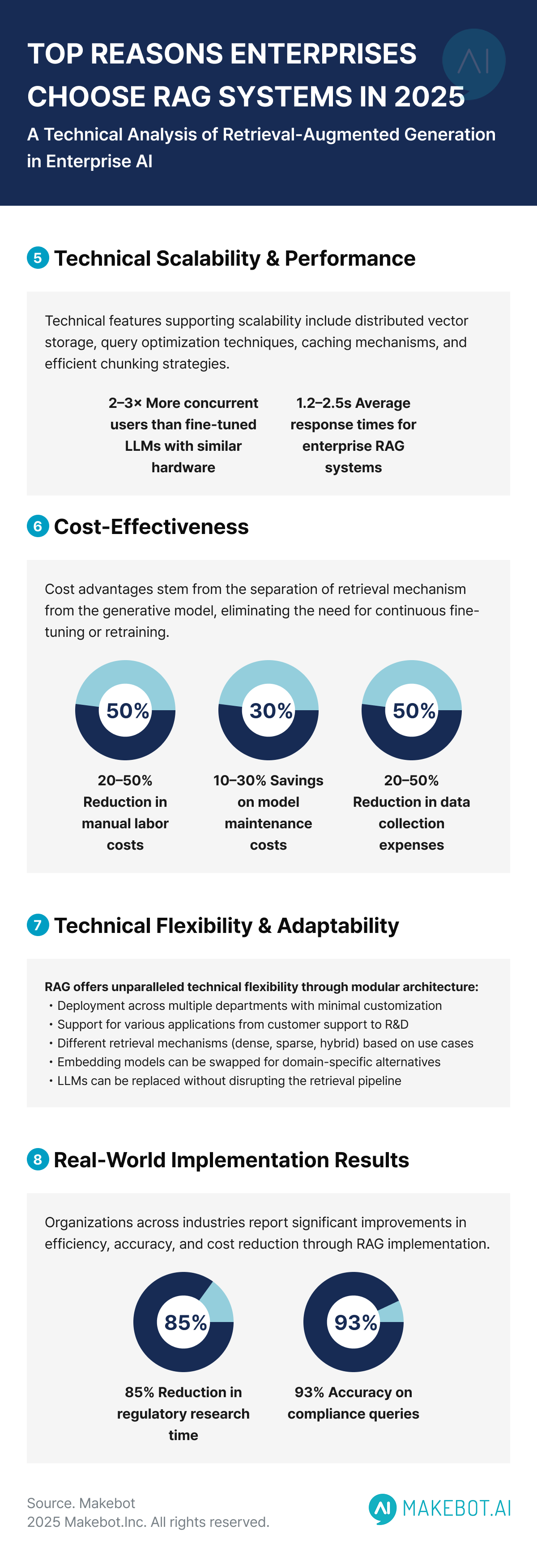
5. Technical Scalability and Performance Optimization
From an engineering perspective, RAG Systems offer superior scalability compared to alternative AI implementation approaches.
- Benchmarks show that RAG architectures can handle 2-3x more concurrent users than fine-tuned LLMs with similar hardware requirements
- Response times for RAG in Enterprise settings average 1.2-2.5 seconds, comparable to or faster than fine-tuned models for complex queries
- Vector database optimization techniques enable efficient scaling to billions of documents while maintaining retrieval performance
Technical features supporting this scalability include:
- Distributed vector storage and retrieval
- Query optimization through techniques like Maximal Marginal Relevance (MMR)
- Caching mechanisms for frequently accessed documents
- Load balancing across retrieval and generation components
- Efficient chunking strategies that optimize retrieval precision
6. Cost-Effectiveness and Resource Optimization
RAG Systems present a compelling technical case for resource efficiency compared to alternative approaches.
- Traditional AI implementations require frequent retraining, consuming significant computational resources
- RAG reduces manual labor costs by 20-50% by automating information retrieval processes
- 10-30% savings on model maintenance costs since RAG eliminates the need for continuous model updates
- 20-50% reduction in data collection and annotation expenses through leveraging existing enterprise documentation
This cost advantage stems from the architectural design of RAG, which separates the retrieval mechanism from the generative model. This separation allows enterprises to benefit from the latest advancements in LLMs without the overhead of continuous fine-tuning or retraining.
7. Technical Flexibility and Adaptability
RAG in Enterprise environments offers unparalleled technical flexibility, enabling businesses to adapt their AI systems to evolving requirements.
- RAG Systems can be deployed across multiple departments with minimal customization
- The same RAG architecture can support various applications from customer support to research and development
- Different retrieval mechanisms (dense, sparse, or hybrid) can be implemented based on specific use cases
- Retrieved contexts can be adjusted in size and scope to optimize for different types of queries
This flexibility is achieved through modular architecture that allows components to be updated or replaced independently:
- Embedding models can be swapped for domain-specific alternatives
- Vector databases can be optimized for specific retrieval patterns
- Chunking strategies can be tailored to different document types
- Prompting techniques can be customized for different use cases
- LLMs can be replaced with newer versions without disrupting the retrieval pipeline
8. Enterprise Implementation: Real-World Results
Case studies from various industries demonstrate the technical effectiveness of RAG Systems:
Financial Services
- 85% reduction in time spent researching regulatory requirements
- 93% accuracy on complex compliance queries
- 67% decrease in compliance-related errors
- $4.2M annual savings in compliance research costs
Healthcare
- 72% faster access to relevant clinical information
- 35% reduction in treatment decision time
- 91% of clinicians reported improved confidence in decisions
- 28% increase in rare condition identification through comprehensive knowledge access
Customer Support
- Call handling times reduced by approximately 25%
- First-contact resolution rates improved by 30-45%
- Overall operational costs decreased by 30-40%
- Customer satisfaction increased due to more accurate and contextually relevant responses
9. Technical Architecture Considerations for Enterprise RAG Implementation
Successful RAG in Enterprise deployment requires careful consideration of several technical components:
Document Processing Pipeline
- Document chunking strategies significantly impact retrieval quality (semantic chunking improves relevance by 25-40%)
- Metadata extraction enhances filtering and relevance scoring
- Pre-processing techniques like OCR and table extraction maintain information integrity
Vector Database Selection
- Trade-offs between recall and latency influence index selection (HNSW, PQ, ANNOY, etc.)
- Storage requirements scale linearly with embedding dimension
- Hybrid search combining dense and sparse methods improves overall retrieval quality by 30-50%
Query Processing
- Query rewriting techniques enhance retrieval precision
- Subquery generation helps with complex information needs
- Relevance scoring algorithms prioritize the most applicable documents
Monitoring and Evaluation
- Comprehensive evaluation frameworks measure retrieval precision
- Real-time monitoring of system performance identifies issues
- User feedback loops enable continuous improvement
How is RAG used in Generative AI? Read more here!
Conclusion: The Technical Case for RAG in Enterprise AI
The adoption of Retrieval-Augmented Generation (RAG) represents a strategic technical decision for enterprises seeking to maximize the value of their AI investments. By combining the linguistic capabilities of Large Language Models (LLMs) with the accuracy and relevance of enterprise-specific knowledge bases, RAG Systems deliver AI solutions that are more accurate, trustworthy, and valuable for business applications.
As AI technology continues to evolve, RAG provides a balanced architectural approach that maximizes the benefits of generative AI while mitigating risks related to hallucinations, outdated information, and irrelevant responses. For enterprises navigating the complex landscape of AI implementation in 2025, RAG AI offers a technically sound path forward that delivers immediate business value while establishing a foundation for future innovation.
Transform Your Enterprise with Advanced RAG Technology
Why leading organizations choose RAG systems in 2025:
MAKEBOT: Enterprise RAG Solutions with Proven Results
Makebot's patent-protected Hybrid RAG technology delivers what matters most:
- 70-90% reduction in AI hallucinations through grounding in verified enterprise knowledge
- 95-99% accuracy on queries about your latest policies and real-time information
- Advanced security features keeping sensitive data within enterprise boundaries
- Hybrid search combining dense and sparse methods for 30-50% better retrieval quality
Industry-Specific Impact:
- Financial Services: 85% reduction in regulatory research time
- Healthcare: 72% faster access to clinical information
- Customer Support: 30-45% improved first-contact resolution
Contact us today:
- Email: b2b@makebot.ai
- Website: www.makebot.ai
Over 1,000 clients are already leveraging Makebot's RAG systems for immediate business value.