How Retrieval-Augmented Generation (RAG) Supports Healthcare AI Initiatives
RAG enhances AI in healthcare by improving accuracy, reducing bias & personalizing care.

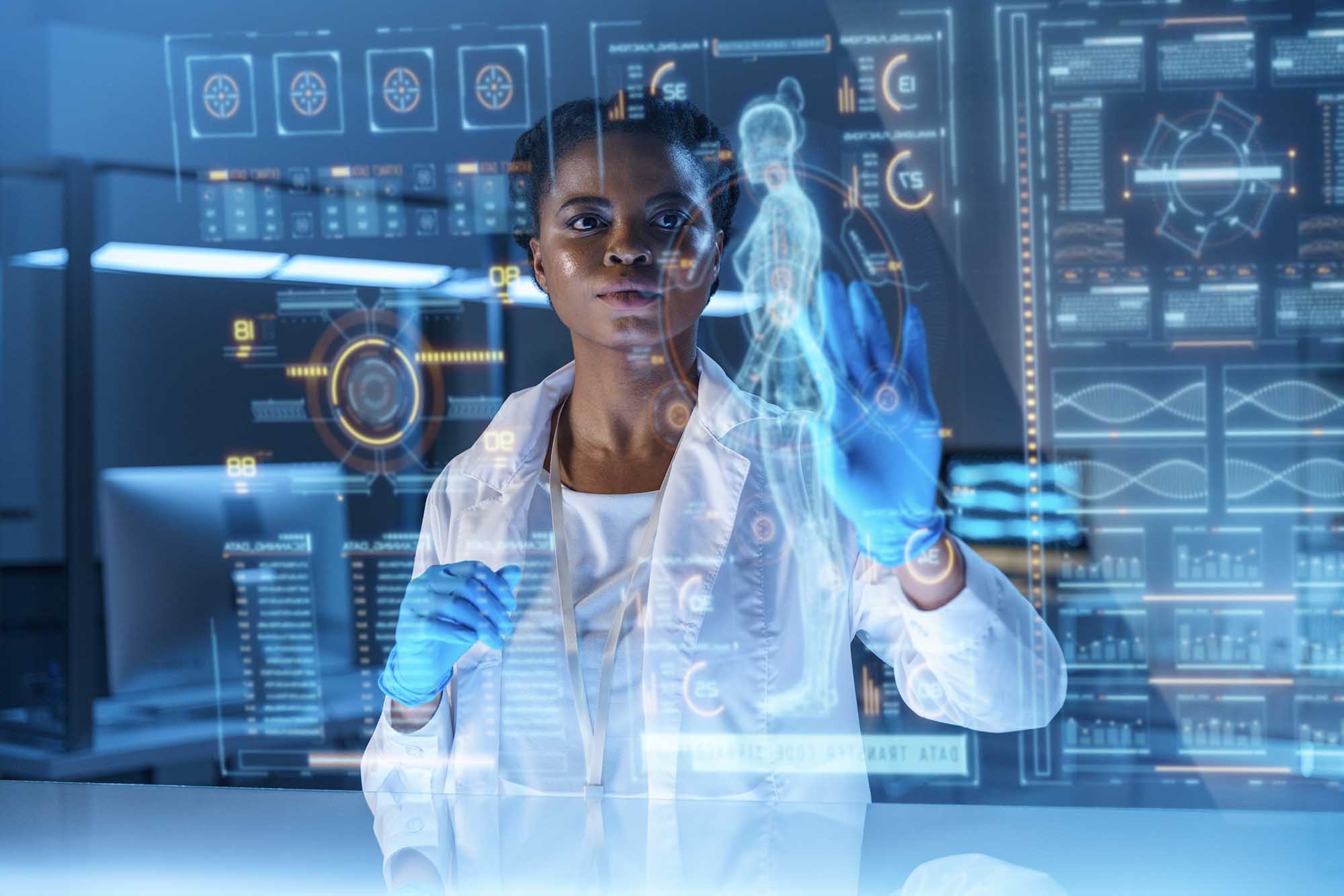
Generative Artificial Intelligence (AI) is transforming healthcare, offering benefits in clinical decision-making, patient education, and administrative efficiency.
However, traditional large language models (LLMs) suffer from biases, inaccuracies, and outdated knowledge, limiting their reliability in high-stakes healthcare environments.
Retrieval-Augmented Generation (RAG) addresses these challenges by integrating real-time, domain-specific knowledge retrieval into generative AI models, improving accuracy, transparency, and personalization in healthcare applications.
Read more about RAG here: How is RAG used in Generative AI
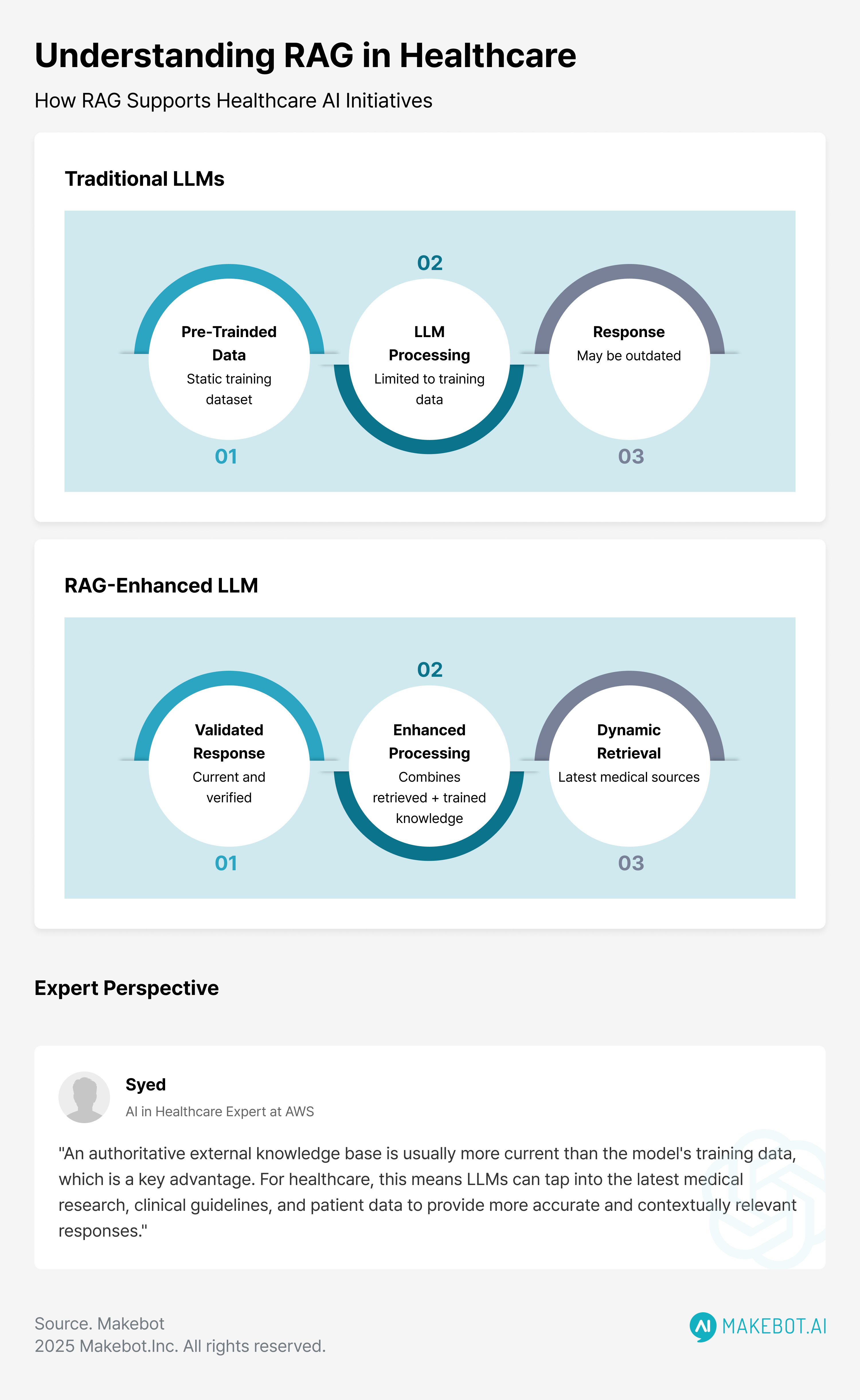
Understanding Retrieval-Augmented Generation (RAG)
RAG is an AI framework that enhances LLMs by retrieving and incorporating external knowledge at runtime.
Unlike traditional LLMs that rely solely on pre-trained datasets, RAG dynamically searches trusted sources such as medical literature, clinical guidelines, and electronic health records (EHRs) before generating a response.
This approach ensures that AI-driven healthcare solutions remain current, precise, and contextually relevant.
According to Syed, an expert of AI in Healthcare at Amazon Web Services, “An authoritative external knowledge base is usually more current than the model’s training data, which is a key advantage. For healthcare, this means LLMs can tap into the latest medical research, clinical guidelines, and patient data to provide more accurate and contextually relevant responses”.
By mitigating the limitations of static training datasets, RAG in healthcare enhances AI-driven decision-making, reduces biases, and improves transparency by linking generated responses to their original sources.
Read Also: The Ultimate Guide to RAG vs Fine-Tuning: Choosing the Right Method for Your LLM
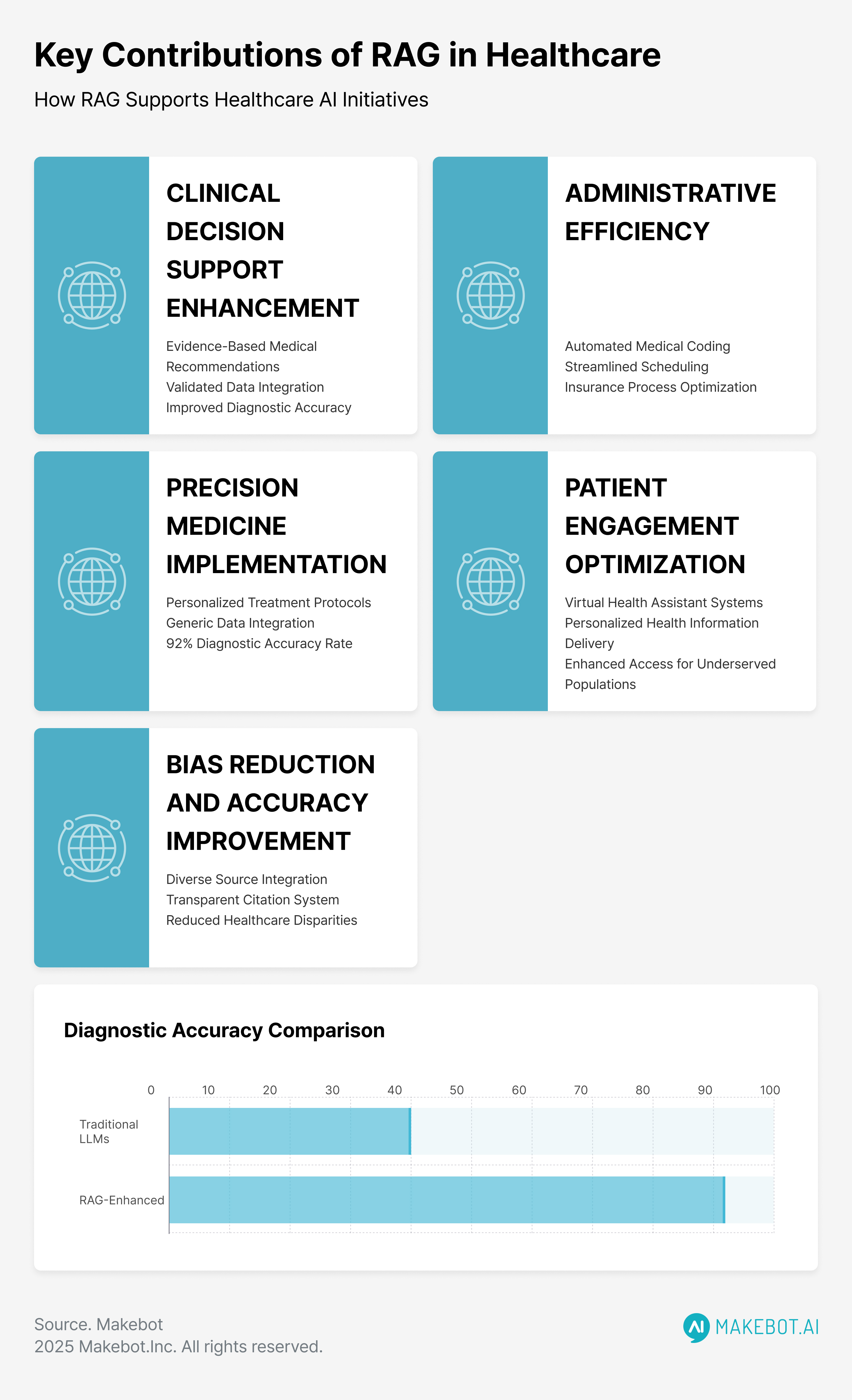
Key Contributions of RAG in Healthcare
1. Enhancing Clinical Decision Support
Medical professionals rely on precise, real-time information to diagnose and treat patients effectively.
Traditional LLMs often generate plausible but incorrect medical recommendations due to a phenomenon known as AI “hallucination.” RAG in healthcare mitigates this issue by retrieving validated medical data from sources such as PubMed, CDC, and NIH before formulating responses.
For instance, in nephrology, a study found that LLMs such as ChatGPT and Google Bard AI had less than 40% accuracy in answering kidney care-related questions. However, when combined with RAG, these models significantly improved their diagnostic precision, ensuring evidence-based recommendations aligned with clinical best practices.
2. Improving Response Accuracy & Reducing Bias
Generative AI models are prone to biases, particularly when trained on datasets lacking representation of diverse patient populations.
This issue can lead to disparities in healthcare recommendations, particularly for minority and underrepresented groups. RAG in healthcare addresses this challenge by retrieving data from diverse, authoritative sources, reducing bias, and promoting health equity.
Additionally, RAG enhances transparency by allowing users to trace AI-generated responses back to the original medical literature or database. This ensures that healthcare professionals can verify AI-generated insights, increasing trust and reliability in clinical decision-making.
3. Personalized Healthcare & Precision Medicine
One of RAG’s most impactful applications is its ability to personalize healthcare recommendations.
By integrating AI-driven healthcare insights with a patient’s medical history, genetic data, and lifestyle factors, RAG enables precision medicine tailored to individual needs.
For example, in chronic kidney disease (CKD) management, a RAG-enhanced AI model retrieved patient-specific clinical guidelines, resulting in more accurate treatment recommendations compared to generic AI models.
This approach significantly improved patient outcomes by ensuring that recommendations were aligned with the latest research and individual patient characteristics.
4. Streamlining Administrative & Operational Efficiency
Beyond clinical applications, RAG in healthcare optimizes workflows by automating administrative processes such as:
- Medical coding: Extracting and summarizing patient records for accurate insurance claims.
- Appointment scheduling: Assisting patients in finding specialists based on their condition.
- Insurance inquiries: Providing real-time updates on coverage and copay details.
A case study from Accolade demonstrated that implementing RAG-powered search reduced response times and improved customer service efficiency in healthcare organizations by consolidating fragmented data sources into a unified AI system.
5. Enhancing Patient Engagement & Communication
Effective patient communication is essential for improving healthcare outcomes. However, traditional AI chatbots often provide generic, sometimes misleading information.
RAG-powered virtual health assistants enhance patient engagement by retrieving personalized health information, answering queries with up-to-date medical guidelines, and explaining treatment plans in patient-friendly language.
A study evaluating a RAG-powered virtual health assistant found that it matched human clinicians in diagnostic accuracy (92%) while significantly improving accessibility for underserved populations.
Want to read more about RAG in other industries? Click here!

Challenges & Considerations in RAG Implementation
1. Data Quality & Validation
The effectiveness of RAG in healthcare depends on the quality of the external data sources it retrieves. If outdated or biased databases are used, the AI-generated recommendations may be flawed. Healthcare organizations must rigorously curate their knowledge repositories to ensure accuracy and reliability.
2. Privacy & Compliance
Given the sensitivity of medical data, integrating RAG in healthcare systems requires strict adherence to HIPAA and GDPR regulations. Organizations must implement robust data governance protocols to protect patient confidentiality and prevent unauthorized access.
3. Integration with Existing Systems
Deploying RAG in healthcare requires seamless integration with EHRs, medical databases, and AI-driven applications. Organizations must invest in interoperability solutions to ensure that RAG-enhanced models can effectively retrieve and process real-time patient data.
4. Managing Bias in Retrieved Data
While RAG reduces biases compared to traditional LLMs, it is not entirely immune to bias if the retrieved medical literature itself contains disparities. AI developers must continuously audit and refine retrieval algorithms to ensure equitable healthcare recommendations.
Future Directions for RAG AI in Healthcare
The future of RAG-powered AI in healthcare includes:
- Seamless EHR integration: Enabling AI to access real-time patient records for enhanced decision-making.
- Predictive analytics: Combining RAG with AI-driven forecasting models to predict disease progression and optimize treatment plans.
- Multilingual medical AI: Expanding RAG capabilities to support healthcare professionals and patients in diverse linguistic and cultural settings.
- Privacy-preserving AI: Developing secure, decentralized RAG models that protect sensitive medical data while enabling real-time retrieval .
Furthermore,
RAG represents a major leap forward in the application of AI in healthcare.
By integrating real-time medical knowledge retrieval into generative models, RAG enhances clinical decision-making, reduces biases, personalized treatment recommendations, and streamlines administrative workflows.
While challenges related to data validation, privacy, and system integration must be addressed, the potential of RAG to improve healthcare efficiency and patient outcomes is undeniable.
As AI continues to evolve, RAG will play a crucial role in ensuring that medical professionals have access to accurate, up-to-date, and trustworthy information, ultimately advancing the future of AI-driven healthcare.
Transform Healthcare AI with Makebot
Boost accuracy, efficiency, and security in healthcare with Makebot’s RAG-powered AI solutions. Our LLM-integrated chatbots provide real-time medical insights, clinical decision support, and personalized patient engagement—all while ensuring HIPAA-compliant data security.
🚀 Why Makebot?
✅ Hybrid RAG for accuracy & reliability
✅ Seamless EHR & data integration
✅ Scalable & cost-optimized AI solutions
✅ Trusted by top healthcare providers
🔗 Learn more: www.makebot.ai/en
📩 Get in touch: b2b@makebot.ai