LLMs in Healthcare 2024: Enhancing, Not Replacing, Doctors
LLMs in Healthcare 2024: Assisting doctors, not replacing them. Discover how.
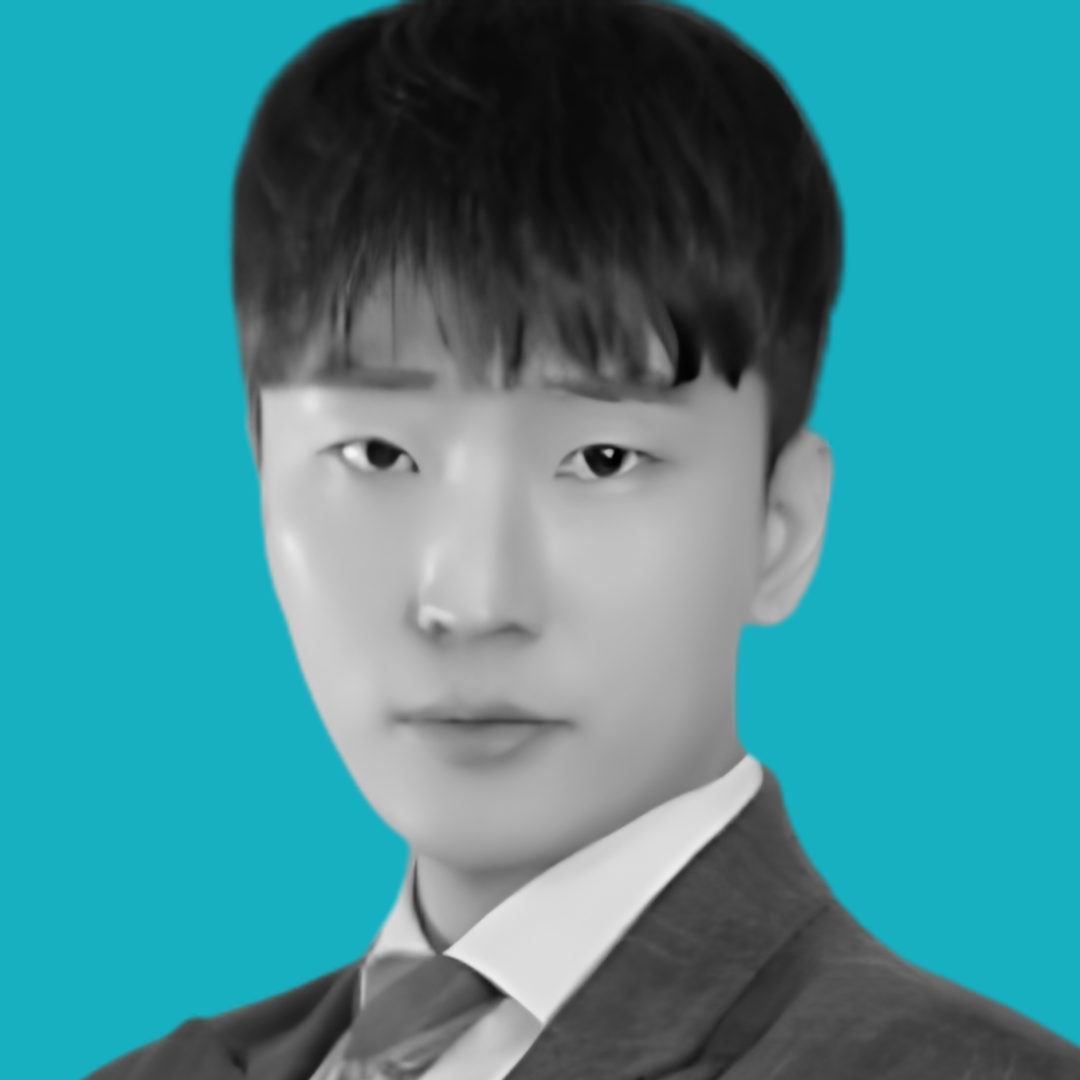

Can AI truly replace human doctors in diagnosing and treating patients, or is it simply an advanced tool to enhance the capabilities of healthcare professionals?
As we move through 2024, the role of artificial intelligence (AI), specifically large language models (LLMs), in healthcare has expanded, challenging the boundaries of traditional medical practices.
But are these AI systems ready to stand on their own, or are they best used to support the critical decision-making of human physicians?
This article delves into the latest studies to explore how AI is reshaping healthcare, highlighting its strengths, limitations, and potential to collaborate with, rather than replace, human doctors.
More on : Doctor AI: The Rise of Generative AI in Healthcare
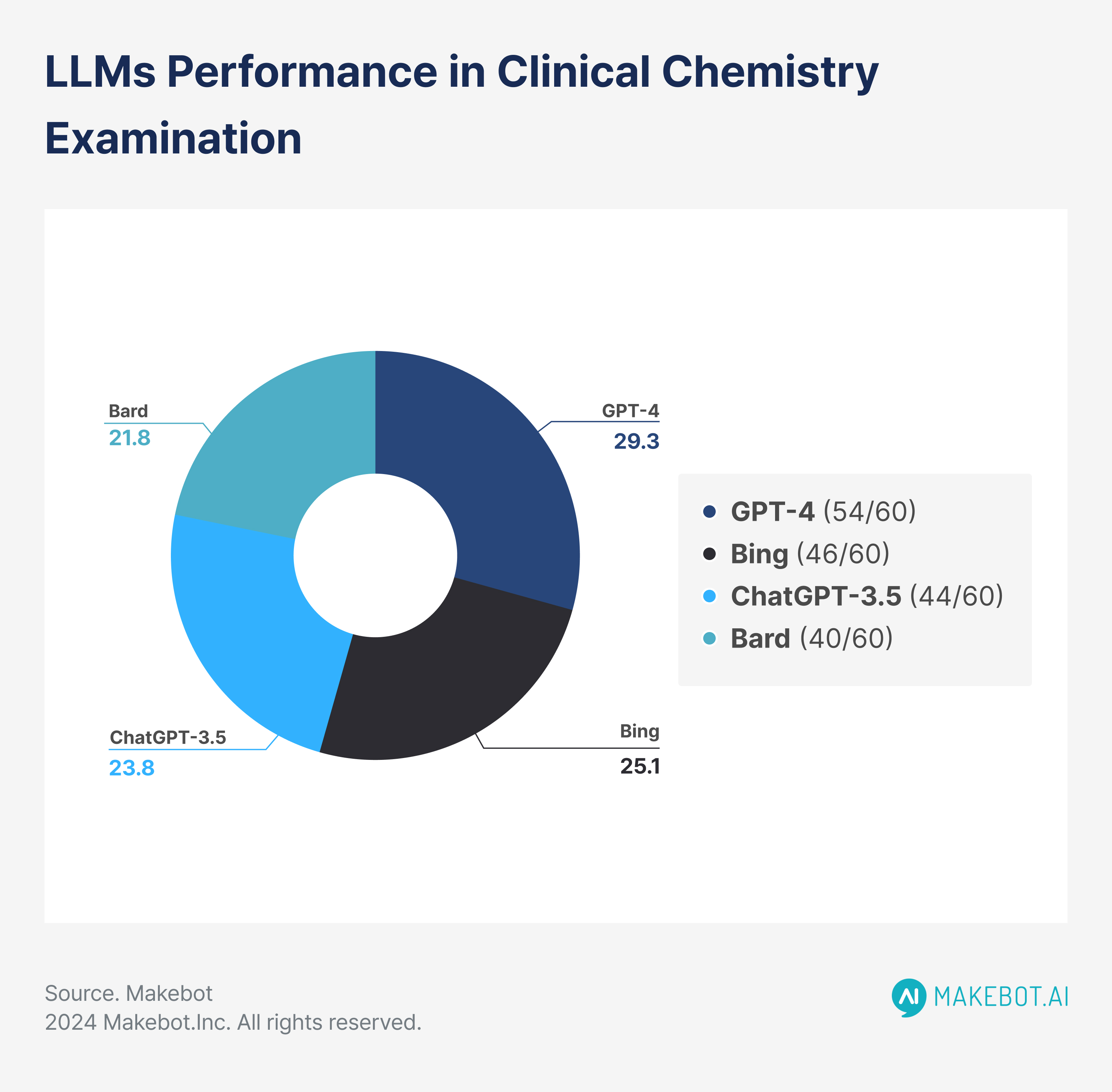
AI Outperforms Humans in Clinical Exams
In a comparative study published by Sallam et al. (2024) in Advances in Medical Education and Practice, ChatGPT-4 was tested against other AI models, including Bing (GPT-4 Turbo), ChatGPT-3.5, and Bard, along with human postgraduate students, in a Clinical Chemistry exam.
- ChatGPT-4 achieved an impressive 90% accuracy (54/60 correct answers), outperforming the human students, whose average score was 66.75%.
- Bing scored 77% (46/60), ChatGPT-3.5 scored 73% (44/60), and Bard scored 67% (40/60), which was closely aligned with the human average.
- 98% of lower cognitive questions (Remember and Understand categories) were answered correctly by ChatGPT-4, but its accuracy dropped to 72% for higher-order questions (Apply and Analyze).
- Humans demonstrated a similar trend, with an average performance of 85% on easier questions but struggled more on complex tasks, scoring 58% in higher-order questions.
This data underscores ChatGPT-4’s ability to excel in knowledge recall but also highlights its struggle with the deeper reasoning required for complex questions, mirroring human performance in some areas.
Read Also: Is Chatgpt Generative AI? (How to Make the Best of it)
.jpeg)
AMIE Outperforms Primary Care Physicians in Diagnostic Accuracy
AMIE (Articulate Medical Intelligence Explorer), developed by Google DeepMind, is an LLM designed to engage in diagnostic dialogue with patients. In a study comparing AMIE to 20 primary care physicians (PCPs) across 149 clinical scenarios, AMIE demonstrated superior diagnostic accuracy in several medical specialties.
- AMIE’s top-3 diagnostic accuracy was 87%, compared to 72% for PCPs. This represents a 15% increase in diagnostic precision for AMIE over human doctors.
- In specialty-specific results:
- Respiratory medicine: AMIE achieved 92%, while PCPs scored 77%.
- Cardiovascular medicine: AMIE achieved 89%, compared to 74% for PCPs.
- Respiratory medicine: AMIE achieved 92%, while PCPs scored 77%.
- In terms of efficiency, both AMIE and the PCPs acquired similar amounts of information from patients during consultations, with an average of 12 conversational turns for AMIE and 11 for PCPs. The number of words elicited from patients was nearly identical—430 for AMIE and 425 for PCPs.
These findings reveal that AMIE is not only more accurate in diagnosing patients but also equally efficient in gathering diagnostic information, making it a valuable tool in clinical settings.
LLMs and Human Communication: A Comparison
The ability to communicate effectively and build trust with patients is an essential aspect of healthcare. In the same study, AMIE was evaluated not only for its diagnostic accuracy but also for its communication skills and patient engagement.
- 96% of patient actors stated they would return to AMIE for future consultations, compared to 88% for PCPs.
- 94% of patients felt confident in the care provided by AMIE, compared to 85% for PCPs.
- Patient clarity: 94% of patients found AMIE's explanations of medical conditions and treatment plans satisfactory, while 85% of patients felt the same about their PCPs.
- Politeness and patient comfort: AMIE scored 95% for politeness and making the patient feel at ease, compared to 87% for PCPs.
These results suggest that LLMs like AMIE can not only match but potentially exceed human doctors in maintaining patient satisfaction and trust through effective communication.

Assessing Medical LLMs
A large-scale study by Xie et al. (2024) at the Shenzhen Research Institute evaluated medical LLMs using two datasets—DoctorFLAN for single-turn queries and DotaBench for multi-turn dialogues.
These datasets, with 91,330 medical instances across 22 tasks and 74 multi-turn conversations, respectively, offered a comprehensive evaluation of AI’s diagnostic capabilities.
- DoctorFLAN: GPT-4 scored an average of 8.42 out of 10 on tasks such as diagnosis, treatment, and medication inquiry. In contrast, medical-specific models like HuatuoGPT and DISC-MedLLM scored 4.29 and 4.24, respectively.
- DotaBench: GPT-4 excelled in handling multi-step reasoning, scoring an average of 9.41, whereas HuatuoGPT trailed at 6.78.
- Fine-tuning improvements: DotaGPT (Baichuan2-7B-Base), a model fine-tuned on the DoctorFLAN dataset, improved its performance by 25.2%, achieving a score of 8.25.
The success of fine-tuned models like DotaGPT highlights the potential for LLMs to become more effective with domain-specific training, especially in complex clinical tasks that require multi-turn interactions.
Estimating Diagnostic Probabilities
A study published in JAMA Network Open (Rodman et al., 2023) compared LLMs to human clinicians in estimating diagnostic probabilities for five clinical cases, including pneumonia, breast cancer, and cardiac ischemia.
- Pneumonia case: The LLM’s median pretest probability estimate was 72% (IQR, 69%-78%), compared to 80% (IQR, 75%-90%) for clinicians. The LLM’s mean absolute error (MAE) was 39.5, compared to 47.3 for clinicians.
- Breast cancer case: After a negative test result, the LLM estimated a posttest probability of 0.2% (IQR, 0.06%-0.3%), outperforming clinicians, who estimated 5% (IQR, 1%-10%). The LLM’s MAE was 0.2, compared to 11.2 for clinicians.
- Asymptomatic bacteriuria: The LLM's pretest probability estimate was 26% (IQR, 20%-30%), whereas human clinicians estimated 20% (IQR, 10%-50%).
In most cases, the LLM outperformed human clinicians in adjusting probabilities after negative test results, suggesting its utility in improving diagnostic accuracy.
However, the LLM performed less effectively after positive test results, where human judgment and expertise still proved superior.
Ensemble Techniques: A Solution for Overcoming AI and Human Limitations
Both LLMs and doctors face limitations in diagnostic accuracy, particularly when it comes to overconfidence. Xavier Amatriain (2023) suggested using ensemble techniques to overcome this, both for AI models and in human practice.
- Ensemble techniques: When combining the opinions of multiple doctors, diagnostic accuracy can increase to 85%, up from the typical range of 55%-60% seen in individual diagnostics. Similarly, querying an LLM multiple times or using multiple models can yield more reliable diagnostic outputs.
- Nvidia’s Guardrails Toolkit: This AI tool uses ensemble techniques to reduce hallucinations and errors, ensuring that AI-generated responses are more accurate.
Ensemble techniques represent a promising solution to improving diagnostic accuracy, whether applied to human clinicians or AI tools, by combining multiple perspectives to create more reliable diagnoses.
Augmenting, Not Replacing, Doctors
While large language models like ChatGPT-4, AMIE, and DotaGPT have demonstrated remarkable abilities in diagnostic accuracy, communication, and probabilistic reasoning, they are not yet at a point where they can fully replace doctors.
Instead, these tools offer the potential to augment human expertise, providing clinicians with more accurate diagnostics, improving patient communication, and enhancing overall care efficiency.
As AI continues to evolve, its integration into healthcare will require careful oversight, rigorous testing, and fine-tuning to ensure that LLMs serve as valuable assistants rather than replacements in the medical field.
For inquiries regarding the Development of Large Language Models (LLMs), please contact Makebot.ai.